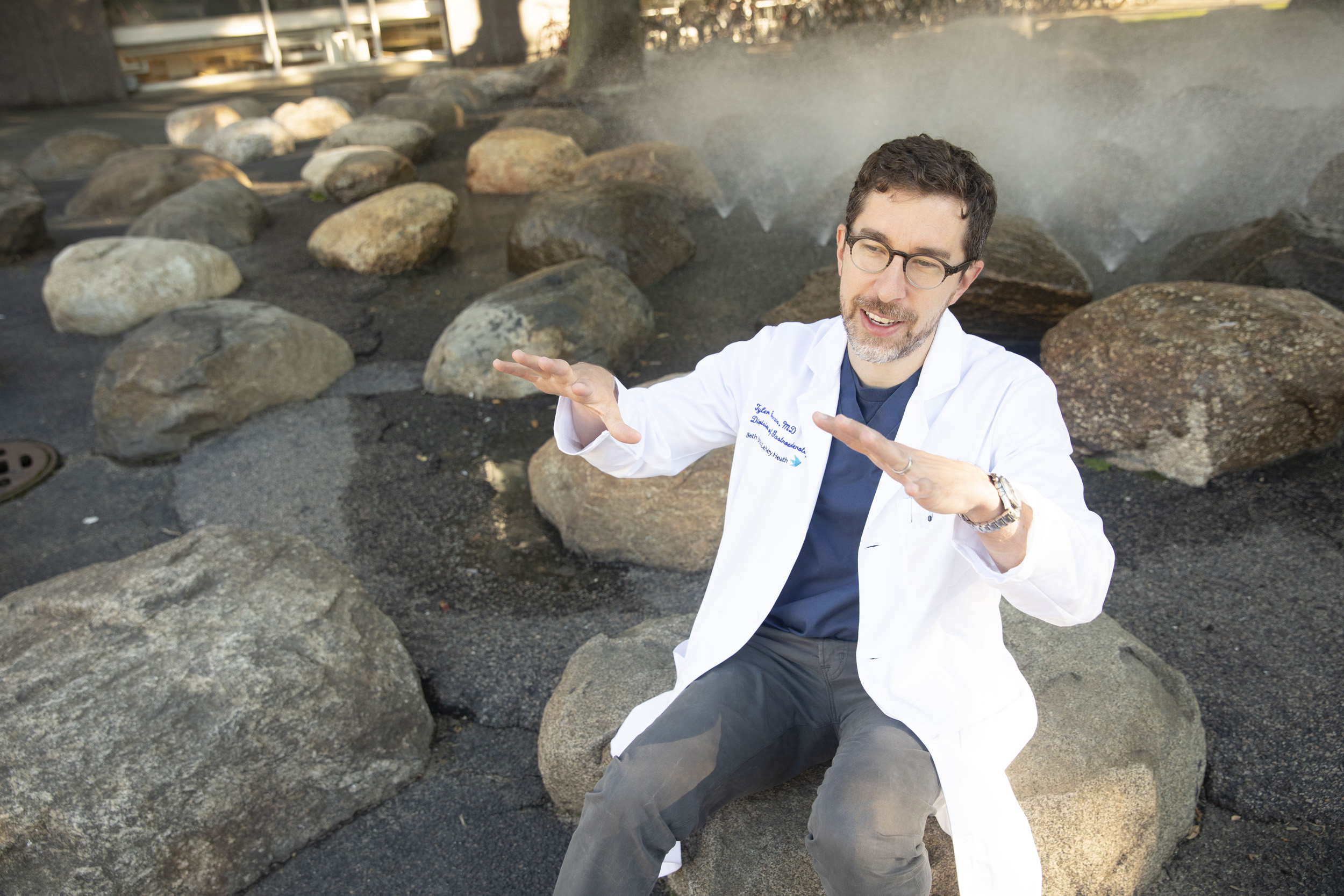
Tyler Berzin’s new study shows that “physicians were about 30 percent less likely to miss a polyp if they were using AI assistance,” the gastroenterologist explains.
Kris Snibbe/Harvard Staff Photographer
Deploying AI against colon cancer
Gastroenterologist explains how algorithm can support physicians, improve accuracy of screenings
Colonoscopy is an important weapon in the fight against colon cancer, which killed 51,000 Americans in 2019, making it the nation’s second-deadliest cancer. But doctors’ ability to catch polyps on the colonoscopy screen varies — sometimes significantly. Last month, a team led by physicians at Beth Israel Deaconess Medical Center and Harvard Medical School showed that an AI-based computer-vision algorithm can improve the accuracy of screenings. The Gazette spoke with Tyler Berzin, a gastroenterologist and associate professor of medicine, about the findings, published in the journal Clinical Gastroenterology and Hepatology. The interview was edited for clarity and length.
Q&A
Tyler Berzin
GAZETTE: Your study used AI to improve colonoscopy results. Can you tell us what you found?
BERZIN: Colonoscopy is the most effective tool for preventing colon cancer, but there’s a lot of variability among individual physicians in their ability to detect precancerous polyps. That variability directly translates into how effectively they can protect their patients from colon cancer. There have been interesting observations in the field of screening colonoscopy that an extra pair of eyes, an experienced nurse or technician, a second gastroenterologist, or an extra gastroenterology trainee helps with polyp detection. So this is a good target for using AI to augment physician performance because AI computer vision could act as an extra set of eyes, without distraction and without fatigue.
But demonstrating real clinical benefit is the last-mile problem for AI in clinical medicine. There is an explosion of very cool technology where AI promises to benefit physician performance and clinical care, but actually demonstrating a benefit in a high-quality randomized control trial has rarely happened. So, our clinical research is providing rock-solid clinical science to support the use of this technology.
GAZETTE: Does the AI review these images after the fact or is it done in real time?
BERZIN: This is real-time use of AI, which also is somewhat unique. In clinical medicine, most examples of AI use — for radiology, for EKGs and so on — is applying AI after the initial patient interaction, in the subsequent review of the X-ray, for instance. But we need real-time assistance during colonoscopy screening, when the job of the physician is to visually survey the entire lining of the colon very meticulously, to identify and remove small precancerous polyps. The challenge that gastroenterologists face is that many of these polyps are very flat, almost growing like moss on a rock. Often they can blend into the surrounding mucosa. Our AI computer sits between the colonoscope and the endoscopy monitor and processes the colonoscopy image. What we see on the monitor is our live colonoscopy procedure, but with blue or green alert boxes pointing out where suspected polyps may be located. It basically guides the physician’s eyes to an area where these subtle polyps are. So this is the perfect example of AI not replacing the physician, but augmenting physician performance.
GAZETTE: Are flat polyps less dangerous?
BERZIN: It’s actually the reverse. These flat polyps, which are often on the right side of the colon, make up a large percentage of polyps that may be missed during colonoscopies. There is a small percentage of patients who develop colon cancers even after they’ve undergone the screening and those patients often are found to have colon cancers in the right side of the colon.
GAZETTE: And the detection improvement was about 30 percent?
BERZIN: Percentages are always tricky, because there’s absolute versus relative differences for any given polyp, but our study showed that physicians were about 30 percent less likely to miss a polyp if they were using AI assistance. A core priority for AI in clinical medicine is independent, external validation of AI clinical algorithms — does an algorithm which has been developed in one environment perform as expected in a different clinical setting with a different patient population? This study is the first prospective randomized trial to externally validate the performance of an AI algorithm in a country and patient population — the U.S. — that was entirely distinct from where the training data was derived, China. We’re particularly proud that the trial engaged a diverse U.S. patient population, which must be a continued priority for AI clinical trials going forward.
GAZETTE: How does the AI recognize images of the polyps?
BERZIN: In this case the software is based on a deep-learning computer vision algorithm — which is built to learn how to detect certain objects once you give it enough examples of what that object looks like. You feed it a lot of visual data and say, “Hey, these 100,000 images have polyps, and these 100,000 images don’t.” Then it learns, over a training period, how to distinguish the polyps. What’s interesting is that these AI deep-learning systems potentially identify features that a physician might not even recognize. There are lots of examples of this, where a deep-learning model for X-rays, for instance, can distinguish somebody’s ethnicity based on an X-ray. There are examples where AI systems can look at a retinal scan and distinguish whether the patient is male or female, which physicians cannot do by looking at the retina. We have no idea how the AI systems can do this, but they can do it with incredible accuracy. So when you train a machine-learning model, it may actually be picking up on cues that are not the typical cues that physicians pick up on. That can come with advantages, but it can also — outside of polyp detection — create interesting questions about what’s happening and why.
GAZETTE: Can the human physicians learn from the AI?
BERZIN: One area of interest is “explainable AI.” We would love to be able to go back and interrogate the computer: Hey, this is an interesting group of 20 polyps that the machine saw more easily than the physician — what are the features that made it possible to reliably identify these and that will help, both with training physicians and with future iterations of the AI technology?
GAZETTE: How long before AI is routinely used in colonoscopies?
BERZIN: The FDA just approved the first AI system for polyp detection, and this is beginning to be rolled out to a handful of centers across the country. However, in the field of medicine it’s common that exciting new technology gets rolled out, and sometimes even gains wide adoption, before high-quality research trials determine whether or not the clinical benefit is clear, and whether the cost is warranted. My team is trying to make sure that we develop a solid evidence base of high-quality research to guide clinical use of AI in gastroenterology.
GAZETTE: I heard at least one person familiar with AI in medicine say that the AI used in social media platforms — basically on his kid’s phone — is far more sophisticated than what is used in medicine these days. Do you share that observation?
BERZIN: I do share that observation. I’ve been working on the concept of AI polyp detection now for about seven or eight years — this was around the time that Facebook started recognizing my face and my sister’s face and my wife’s face on our uploaded images on Facebook. Facial recognition is a very difficult problem and tightly parallels some of the image recognition challenges in medicine. Social media companies invested in this years ago, but in the early days of AI, the Venn diagram of people who were interested in machine learning and the folks who were interested in colorectal cancer prevention had almost zero overlap. When I was doing very early research in this eight years ago, several members of my team — all graduate students or postdocs at MIT — ultimately graduated and left for Facebook, Google, or a similar company.
GAZETTE: How big is the missed opportunity?
BERZIN: I think we’re five to eight years behind where we could be if the efforts of the best AI minds had been spent differently during the last decade. Implementing these technologies in medical practice is going to reduce the number of people who develop colon cancer. It’s great that it’s happening in 2021, but certainly I would have been really happy for this to be happening in 2015.