Students use computational biology to confront COVID-19
Creating way to jump-start the immune system’s battle against the disease
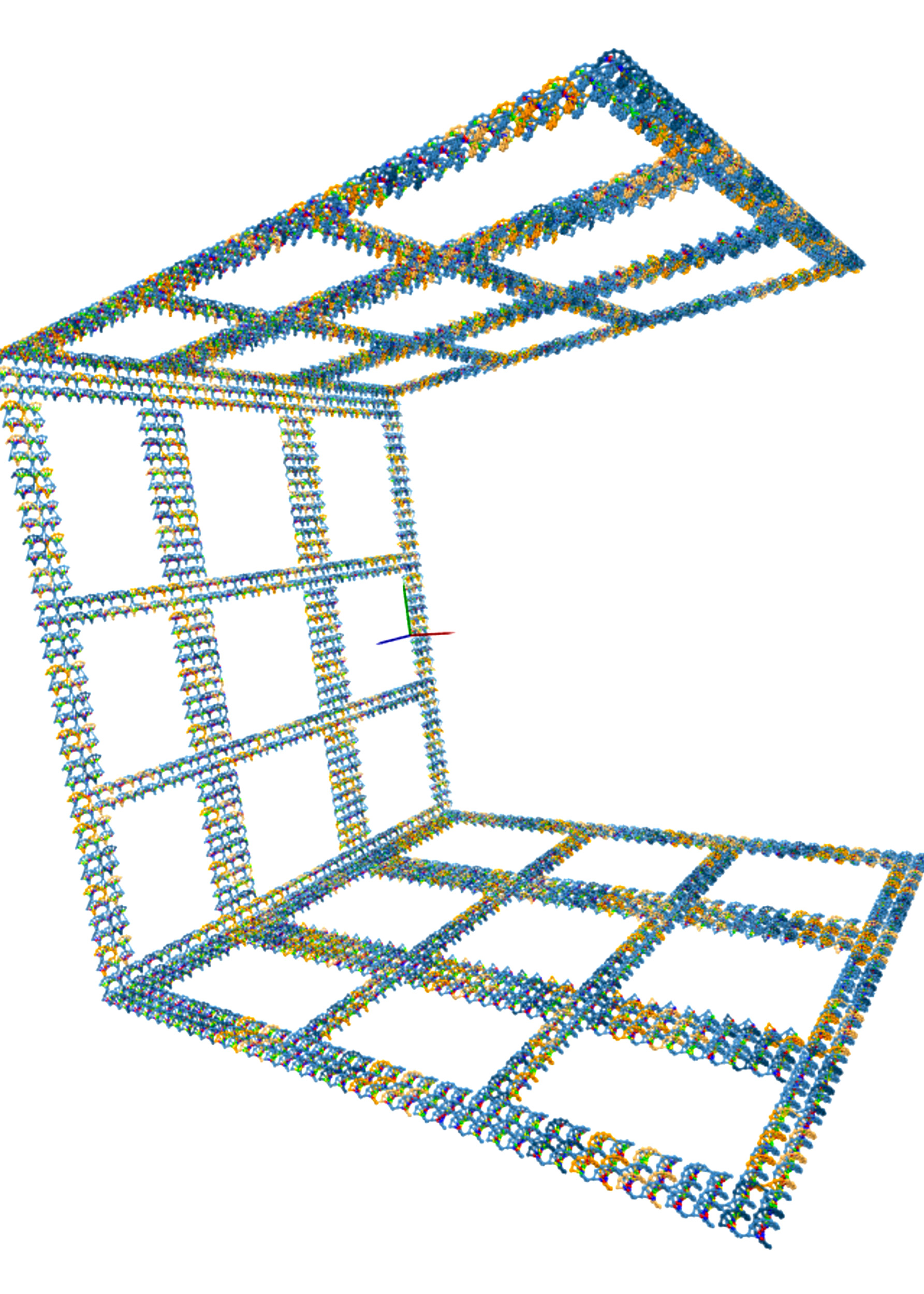
A 3D model of the DNA microstructure the iGEM team is building using computational biology techniques.
Image provided by the Harvard iGEM team
Six months into the global pandemic, there is still no specific antiviral treatment for COVID-19. A team of Harvard undergraduates is working to change that.
Members of Harvard’s International Genetically Engineered Machine (iGEM) team are using computational biology to develop a therapy that enables the body to quickly develop COVID-19 antibodies and jump-start the immune system’s battle against the disease.
“Our goal is to make a viral therapeutic that is easily accessible to people across a wide range of socioeconomic backgrounds, and is also quick to produce,” said Frank D’Agostino ’23, an applied math concentrator. “As we’ve seen, with lockdowns there aren’t easy ways to get into labs. So to be able to do things computationally is crucial because it reduces the amount of time you have to spend in the lab.”
Using computational tools, and collaborating via Zoom and Slack, the students are designing a DNA origami structure to deliver a COVID-19 antibody protein directly to the immune system.
Building the origami structure involves folding DNA strands into specific shapes, drawing on different methods of DNA complementarity, to create an effective delivery vehicle for the molecular cargo.
“Our goal is to make a viral therapeutic that is easily accessible to people across a wide range of socioeconomic backgrounds, and is also quick to produce.”
Frank D’Agostino ’23
“The DNA origami nanostructure acts as the capsule to protect the cargo that we want to deliver to the specific cells,” said David Cao ’23, a bioengineering concentrator. “It also acts as the specificity to target which cells, because there are so many cells in the body. The DNA origami has little receptors on the outside of the box that can attach to specific parts of the cells we are trying to target.”
Inside that DNA origami nanostructure will be a tiny sequence of antibody mRNA that will enable the body’s B-cells to produce a burst of COVID-19 antibodies. The students are using machine learning to sift through tens of thousands of different antibodies, then identify and optimize the best one to fight COVID-19, from which they will draw the mRNA.
Utilizing mRNA rather than the antibody itself is significantly less expensive, which could enable this therapy to be more widely adopted, said Robert Shekoyan ’23, a human developmental and regenerative biology concentrator.
Using machine learning has been a learning experience, D’Agostino said, as the team has had to determine which machine learning technique is the right tool for their process — a process that would typically be performed experimentally.
“We are trying to translate one-dimensional sequence data into what will hopefully be a three-dimensional antibody that has to interact in a complex environment,” D’Agostino said. “It has been challenging to get the resources and know-how to translate those things correctly without making major assumptions that could be detrimental to the machine learning process.”
The work has emphasized to the students the critical role of computational biology, and how machine learning and computational design methods will become even more significant for future synthetic biology research.
To adviser Jia Liu, assistant professor of bioengineering at the John A. Paulson School of Engineering and Applied Sciences, the remote nature of this year’s iGEM project highlights the importance of computational training for students in experimental sciences.
He sees this as a golden opportunity to implement more computational biology components into iGEM, combining computational techniques with bench work to show how these cutting-edge tools can enhance experimental research.
And since iGEM is offering teams the option of a two-phase project over the next two years, the 2021 iGEM team may be able to pick up this computational groundwork and bring it into the lab, experimentally validating the effectiveness of the antibody design and DNA structure.
“Things are very different in a virtual setting,” D’Agostino said. “We need to do all this while having the foresight of being in a laboratory setting so we can set ourselves up for success. Everything can be much more complicated in a real-world scenario.”