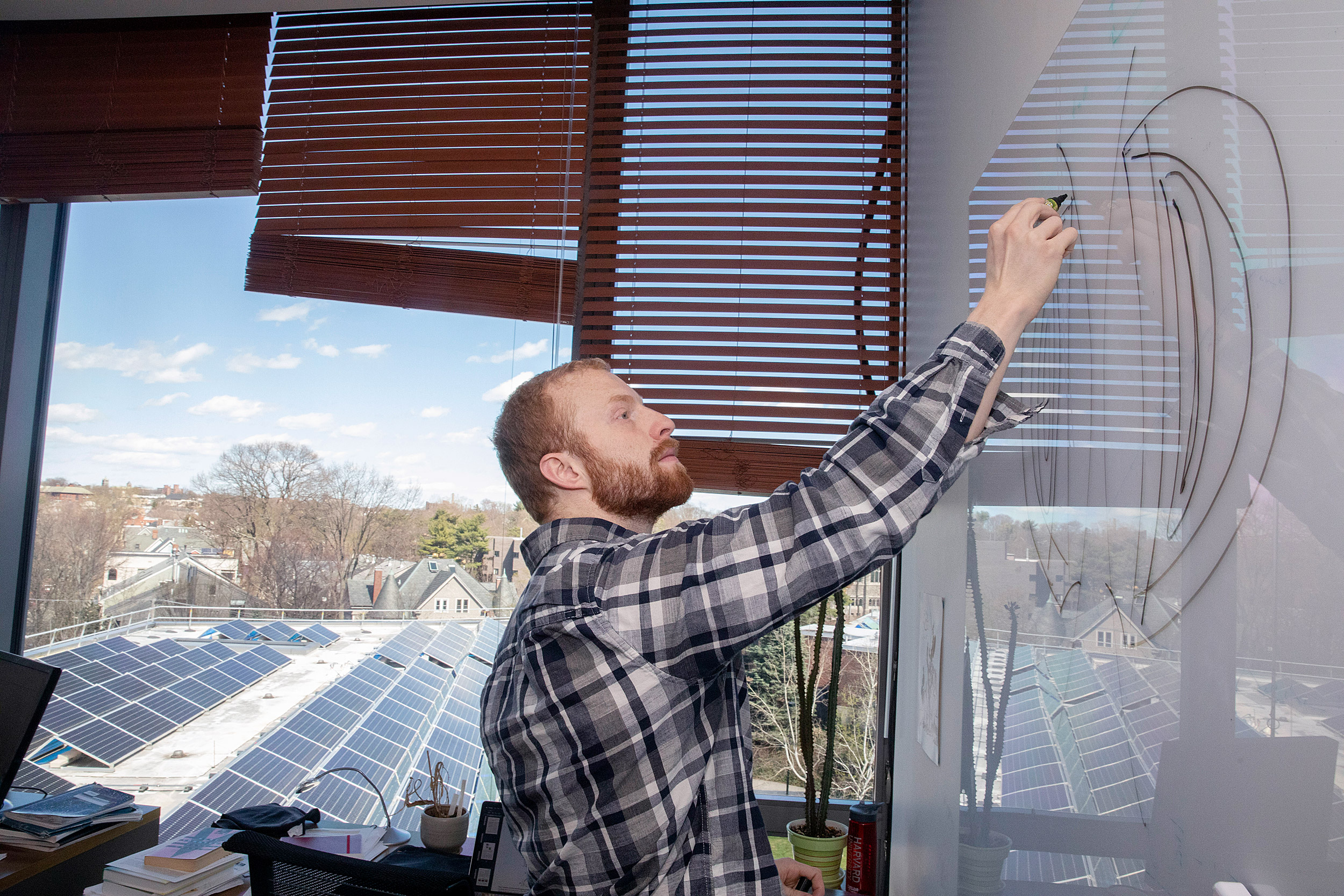
Physics Ph.D. student Julian Kates-Harbeck explains artificial intelligence and fusion energy.
Kris Snibbe/Harvard Staff Photographer
Containing the sun
In the quest for clean, limitless energy through nuclear fusion, scientists use ‘deep learning’ AI to predict destructive disruptions
For decades, scientists have been trying to develop clean, limitless energy by re-creating the conditions at the center of the sun here on Earth. But if nuclear fusion is to be practical for electricity production, that immense, raging power must be controlled.
“When the plasma in a fusion experiment becomes unstable, it can escape confinement and touch the wall of the machine, causing severe damage and sometimes even melting or vaporizing components,” said Julian Kates-Harbeck, a physics Ph.D. student and a Department of Energy (DOE) Computational Science Graduate Fellow. “If you could predict those escapes, or ‘disruptions,’ you could mitigate their effects and build in safety protocols that would cool the plasma down gently and keep it from damaging the machine.”
In a new study published in Nature and led by the U.S. DOE’s Princeton Plasma Physics Laboratory (PPPL), Kates-Harbeck and his colleagues created a “deep learning” artificial intelligence (AI) code to successfully forecast disruptions in fusion reactors. The new method’s predictions can be applied across machines, making it a major step forward for international fusion energy initiatives.
The harder they come, the harder they fall
In the sun, lighter elements are fused into heavier ones in the form of intensely hot plasma, which generates energy. To re-create fusion, scientists use a tokamak: a building-sized, donut-shaped machine that contains hot plasma using a powerful magnetic field.
“Some of the biggest nuclear fusion machines in the field — hundreds of tons of solid steel — can jump a centimeter up in the air when a disruption happens,” said Kates-Harbeck. “That gives you an idea how much power is released. You really don’t want this to happen.”
Disruption prediction is critical, because the bigger the machine, the bigger the disruption. In the $25 billion Iter tokamak currently under construction in France, disruptions are expected to be severe: The machine has more than eight times the volume and energy of the Joint European Torus (JET), the next-largest magnetically confined plasma physics experiment in operation, and less surface area to capture it.
“We don’t have good strategies for completely avoiding these disruption events yet,” said Kates-Harbeck. “The best thing we can do is to predict when they are going to happen so we can avoid most of their adverse effects. That might be, for example, by injecting neutral gas that cools the plasma before it smashes into the wall. But you can’t mitigate anything if you don’t know it’s coming.”
Summer research
Kates-Harbeck worked at Princeton for a summer through his DOE fellowship. There, he teamed up with Bill Tang, principal research physicist at PPPL, professor in the Department of Astrophysical Sciences at Princeton, and the senior author on the study. Tang was just starting a new project using machine learning to tackle disruption prediction. For Kates-Harbeck, it was the perfect combination.
“My background is in physics and AI, so being able to combine both while working on a problem as meaningful as fusion energy — a topic I had always wanted to work on — was like hitting the jackpot,” he said. “People have been using classical machine learning on disruption prediction for years, but I’d just come out of my [computer science] master’s program and got really excited about applying deep learning to the problem. Bill was very open and supportive of the idea, and that’s how we got started.”
Tang called artificial intelligence “the most intriguing area of scientific growth right now,” and said, “to marry it to fusion science is very exciting. We’ve accelerated the ability to predict with high accuracy the most dangerous challenge to clean fusion energy.”
A CC-BY image of a linear fusion device.
Courtesy of EUROfusion
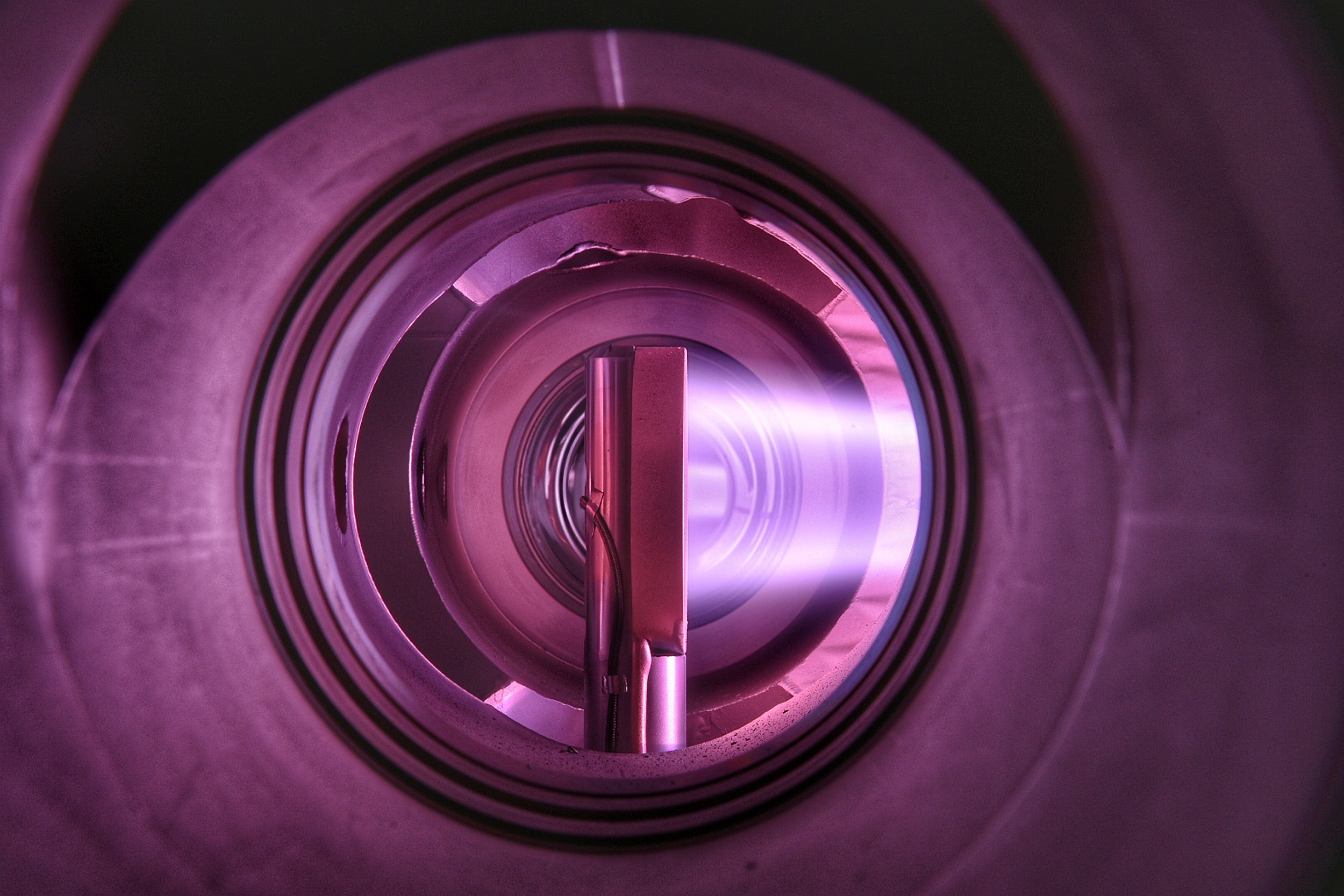
Deep-learning AI
Machine learning needs to be trained in order to learn. To train their code, the team used massive, diverse streams of measurement data from past experiments. Their new algorithm, the Fusion Recurrent Neural Network (FRNN), searches for patterns in the data that tend to occur before a disruption happens. FRNN learns these patterns, which allows it to make disruption forecasts.
This is not the first study to apply AI to disruption prediction, but FRNN was based on deep learning, which made all the difference. Deep learning is a distinct field in AI that can handle much more complexity than other approaches.
“Classical machine learning is good at analyzing simple sources of data, such as the average density or current in the plasma,” said Kates-Harbeck. “But we have access to data that is much more complex, like the temperature of electrons as a function of radius in the plasma. That’s high-dimensional data, and you need deep learning to make sense of it.”
High-dimensional data may contain much more information about what is going on in the plasma. That is what allows the deep-learning algorithm to make predictions so much better than other AI methods.
Given enough data, deep learning has a lot of generalization power; that is, what it learns in one structure can be applied in another. Generalization is critical for fusion prediction, where disruptions get worse in larger machines.
Scaling up
The Iter nuclear fusion experiment is a collaboration between 35 countries. In the coming decades, scientists believe the plasmas in the reactor will move well beyond the energy break-even point — the moment when it releases at least as much energy as is required to heat it.
At 23,000 tons and 150 million degrees Celsius, “Iter is going to be so big and powerful that it can’t afford more than a handful of disruptions in its entire lifetime,” said Kates-Harbeck. “But you need thousands of disruptions to train a machine-learning algorithm. That’s why we need to train on one machine that can afford the disruptions, and test the predictor on another.”
Data for the study was provided by two facilities: the DIII-D National Fusion Facility, operated by General Atomics for the DOE in California, and JET in the U.K., managed by the EUROfusion consortium. The team showed that the FRNN code trained on the smaller DIII-D was able to generalize to the larger, much more powerful JET.
This holds great promise for the code’s ability to forecast disruptions on Iter.
“This research opens a promising new chapter in the effort to bring unlimited energy to Earth,” said PPPL Director Steve Cowley. “Artificial intelligence is exploding across the sciences and now it’s beginning to contribute to the worldwide quest for fusion power.”
“This has been a really exciting first step,” said Kates-Harbeck, “but of course simply mitigating an oncoming disruption is a rather crude approach. Next, we’ll need to shift focus from predicting disruptions to actively controlling the plasma in the tokamak. The ultimate goal is to learn how to adjust the plasma to avoid disruptions in the first place.”
Support for this work comes from the Department of Energy Computational Science Graduate Fellowship Program of the DOE Office of Science and National Nuclear Security Administration, Princeton University’s Institute for Computational Science and Engineering, and Laboratory Directed Research and Development funds that PPPL provides.
Source article: Kates-Harbeck J, et al. (2019). Predicting disruptive instabilities in controlled fusion plasmas through deep learning. Nature (in press). Published online 17 April; DOI: 10.1038/s41586-019-1116-4