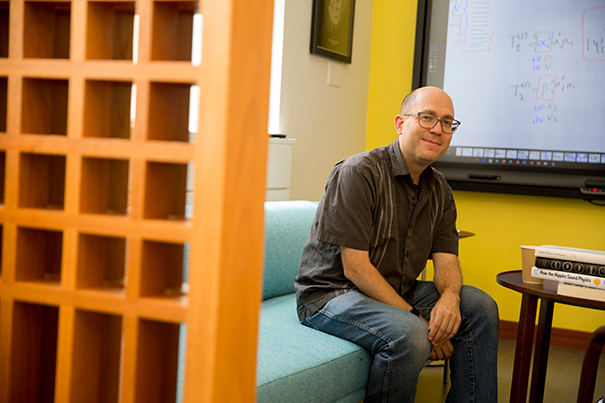
Alan Aspuru-Guzik is among the co-authors of a new study that shows how quantum computers can be used to understand chemistry, a breakthrough that could lead to the development of new materials with unique properties.
Rose Lincoln/Harvard Staff Photographer
New way to model molecules
In study, quantum computer simulates their behavior accurately, which can greatly speed up research
Imagine a future in which hyper-efficient solar panels provide renewable sources of energy, improved water filters quickly remove toxins from drinking water, and the air is scrubbed clean of pollution and greenhouse gases. That could become a reality with the right molecules and materials.
Scientists from Harvard and Google have taken a major step toward making the search for those molecules easier, demonstrating for the first time that a quantum computer could be used to model the electron interactions in a complex molecule. The work is described in a new paper published in the journal Physical Review X by Professor Alán Aspuru-Guzik from the Department of Chemistry and Chemical Biology and several co-authors.
“There are a number of applications that a quantum computer would be useful for: cryptography, machine learning, and certain number-theory problems,” Aspuru-Guzik said. “But one that has always been mentioned, even from the first conceptions of a quantum computer, was to use it to simulate matter. In this case, we use it to simulate chemistry.”
More like this
“There are millions or even trillions of possible molecules,” said Jonathan Romero Fontalvo, a Ph.D. student in Aspuru-Guzik’s lab and one of the lead authors of the study. “If you are an experimentalist hoping to find a new molecule for a drug, you need to consider a huge number of possibilities, and you need to synthesize and test them all. That is extremely costly and requires a great deal of time and effort.”
Classical computers can model simple molecules, but they lack the horsepower needed to model all the possible interactions that take place in more complex molecules.
A molecule like cholesterol, Aspuru-Guzik said, is all but impossible to model exactly in traditional systems because it would require decades to describe how its electrons interact.
Though Aspuru-Guzik and colleagues had described an algorithm to model molecules using quantum computers more than a decade ago, quantum computing resources were limited at the time, meaning the team was only able to test certain parts of the algorithm.
The new study not only marks the first time the entire algorithm has been tested in a scalable manner, but also implements it with a new algorithm, dubbed the variational quantum eigensolver. Even more importantly, Aspuru-Guzik said, both algorithms were implemented in a scalable approach, meaning that while they were tested on a small molecule, they would work equally well on a larger, more complex compound.
“We were actually able to compare our old algorithm against the new one,” he said. “The machine is so powerful we can do that. And because it’s scalable, the same algorithm we would run against any molecule in this case was run against a small molecule.”
Using the algorithm, Aspuru-Guzik and colleagues are able to model the electronic structure of a given molecule, and then to “read” that information, giving them precise data about behavior and interactions.
Armed with that information, he said, researchers can understand whether a molecule will possess the properties desired — whether it will bind to an enzyme or protein, whether it will catalyze certain reactions, and whether a material will possess specific traits.
“This is arguably the most valuable application for a quantum computer,” he continued. “Commercially, the market for fine chemicals is estimated to be worth $3 trillion. A number of other teams, including researchers at Microsoft and national labs, have made this area a priority.”
But without quantum computers, Aspuru-Guzik said, that search would amount to little more than a guessing game.
That is why “I like to call this a disruptive innovation,” he said. “All the methods we have now are approximations, which means if we want to discover a new battery or a better LED, our calculations will sometimes fail. But the change a quantum computer brings is that the answer is exact. Period. That trust will allow us to discover molecules much quicker, make molecules that are much more interesting, and explore chemical spaces significantly faster. When I started at Harvard in 2006, I never imagined that 10 years later we would be at this point.”
The future is likely to bring hardware advances.
“We are currently fabricating a new generation of quantum chips that will enable much larger calculations,” said co-author Ryan Babbush, a quantum engineer at Google. “We are optimistic that these chips will be large enough to model small transition metal complexes, which are notoriously difficult for classical computers.”
The study has implications to other areas of study, such as machine learning.
“I very much like to think of variational quantum algorithms as a quantum generalization of neural networks,” Google co-author Hartmut Neven said. “Naturally we expect quantum neural networks to be more capable than their classical counterparts in describing quantum systems. An interesting open question is whether they will also prove superior in learning classical data.”
Harvard’s Office of Technology Development has filed several patent applications relating to the software that drives Aspuru-Guzik’s quantum computing platforms.
The study was funded by the Luis W. Alvarez Postdoctoral Fellowship in Computing Sciences at Lawrence Berkeley National Laboratory, the Air Force Office of Scientific Research, the Army Research Office, the Office of Naval Research, and the National Science Foundation.