Researchers introduce innovative method to decode complex neural data
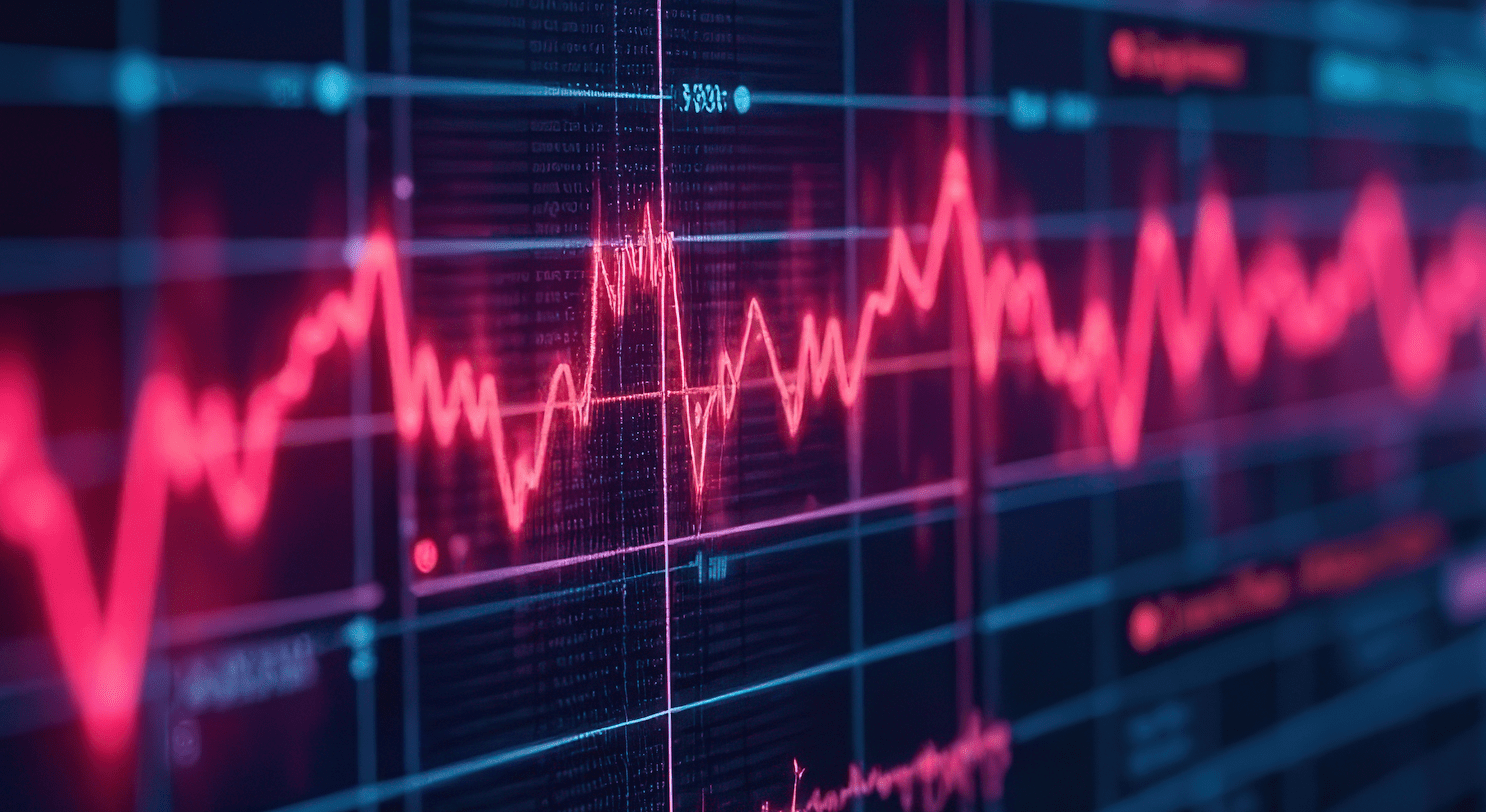
In a new study, researchers introduce a machine learning framework that helps disentangle information patterns in the brain.
Credit: Adobe Stock
A key goal of neuroscience is understanding how the brain processes disparate streams of information from both the external world and from within the body. When neuroscientists measure activity within the brain, these incoming streams of information are often “entangled,” making it difficult to identify what specific inputs a neuron or group of neurons is responding to. “Disentangling” the information in these neural measurements is a key challenge for neuroscientists trying to understand how the brain makes sense of the world.
Artificial intelligence, and specifically machine learning techniques, are allowing scientists to better analyze recordings of brain activity and disentangle neural patterns. A new study, recently published in the journal Neuron and led by Demba Ba, Kempner Institute associate faculty member and Gordon McKay Professor of Electrical Engineering at SEAS, introduces a new machine learning framework for analyzing and disentangling complex neural data.
This new computational framework, called Deconvolutional Unrolled Neural Learning (DUNL), allows scientists to decompose a time series of neural signals into simple elements, allowing scientists to better understand how a neuron, or a population of neurons, responds to external events.