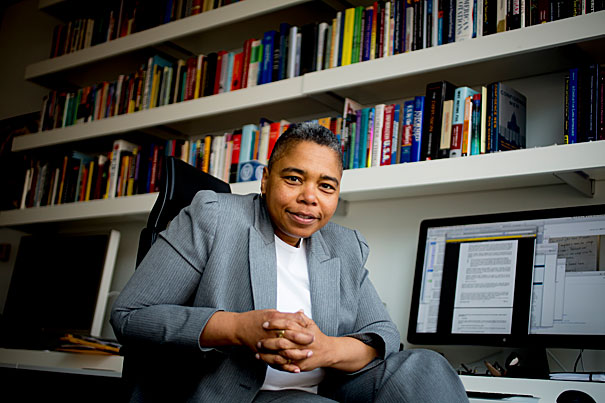
“At the end of the day, online advertising is about discrimination. You don’t want mothers with newborns getting ads for fishing rods, and you don’t want fishermen getting ads for diapers. The question is when does that discrimination cross the line from targeting customers to negatively impacting an entire group of people?” asked Latanya Sweeney, Harvard professor of government and technology in residence, who discovered an advertising bias in Google’s search function.
Rose Lincoln/Harvard Staff Photographer
Seeking fairness in ads
Researcher outlines how ‘bias score’ could be calculated in online delivery
To Internet users, they’re an often-ignored nuisance. But to Google, online ads are both big business and a showcase for some serious mathematics. The determination of which ad ultimately shows up on your computer screen is made by an algorithm that considers everything from how much a company pays for an ad to how well its website is designed.
Latanya Sweeney, Harvard professor of government and technology in residence, wants to add another factor to the mix, one that measures bias. As described in a paper published simultaneously in Queue and Communications of the Association for Computing Machinery, Sweeney described how such a calculation could be built into the ad-delivery algorithm used by Google.
“We’re not trying to get rid of all discrimination,” Sweeney said. “At the end of the day, online advertising is about discrimination. You don’t want mothers with newborns getting ads for fishing rods, and you don’t want fishermen getting ads for diapers. The question is when does that discrimination cross the line from targeting customers to negatively impacting an entire group of people?”
Sweeney discovered one such example. In a paper published earlier this year, she found that Internet searches for “black-sounding” names — such as Darnell or Ebony — were 25 percent more likely to result in the delivery of an ad suggesting that the person had an arrest record, even when no one with the name had an arrest record in the company’s database.
“I was working with a colleague, and we were searching for an old paper of mine,” Sweeney said. “We Googled my name, and up popped an ad suggesting I had an arrest record. I do not, and I was so shocked I spent hours seeing which names got the ads and which did not. I went through the entire study believing I would eventually prove no association with race, but that wasn’t the case.”
Fortunately, she said, the same techniques that uncovered that apparent bias can be used to counteract it. In her paper, Sweeney describes how an algorithm can identify white- or black-sounding names and calculate a “bias score” based on how often negative ads are delivered for that name.
If, for example, someone were to search for “Latanya Sweeney,” the algorithm could determine whether a disproportionate number of ads offering to search for an arrest record have been delivered in the past, and counteract that bias by discounting the ad to encourage the delivery of more neutral ads.
The roadmap for making such calculations, Sweeney said, was laid out in an earlier paper of hers.
In that study, Sweeney explained, she first had to determine what white- and black-sounding names were. To do that, she turned to earlier studies that had collected birth records and tracked the first names given to white and black children. Armed with that data, she conducted thousands of Google searches to produce a list of nearly 2,200 real people who had those first names.
Sweeney then visited two sites, Google.com and Reuters.com, and searched for each name once while recording which ads came up. The results, she said, were startling.
Overall, black-sounding names were 25 percent more likely to produce an ad offering to search for a person’s arrest record. For certain names, like Darnell and Jermaine, the ads came up in as many as 95 percent of searches. White-sounding names, by comparison, produced similar ads in only about 25 percent of searches.
One possible explanation for the phenomenon is that the bias is simply an unintentional side effect of the way Google’s algorithm weights certain ads based on what companies will pay and how often they are clicked on. Whether that is the case or bias is introduced through an advertiser, Sweeney said, the bias measure could easily be built into the algorithm as another weighting factor, controlling for both.
“Google is already weighting some ads more than others,” Sweeney said. “What we’re saying is we want to define another measure, called a bias measure, using the same comparisons we used to identify the bias in our prior study. This could be very easily built into the way Google’s algorithm already works.
“We can now define a predicate for finding this bias,” she said. “In our prior study, that predicate was whether the name being searched was a ‘white’ name or a ‘black’ name, but we could do the same thing with male or female names, or other ethnic groups and other groups protected from specific forms of discrimination by law.”