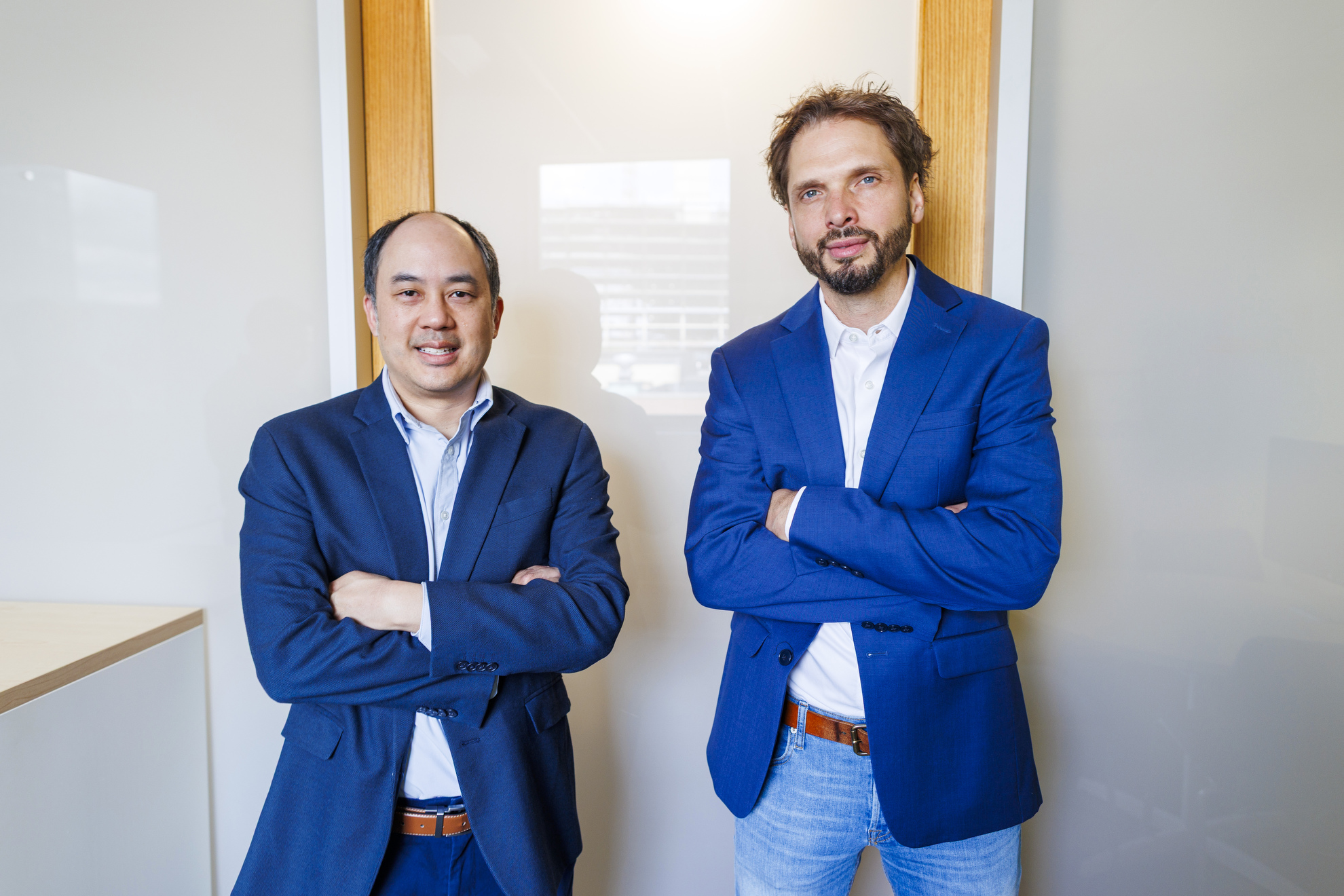
Raymond Mak (left) and Hugo Aerts.
Stephanie Mitchell/Harvard Staff Photographer
New AI tool predicts biological age by looking at a face
Deep-learning algorithm FaceAge uses snapshots, can help oncologists tailor treatments
A new artificial intelligence tool developed by researchers at Mass General Brigham and Harvard Medical School uses a snapshot of a patient’s face to predict biological age and cancer survival time, knowledge that physicians can use to tailor treatments.
“We all know that people age in different ways. A person’s chronological age is based on the day they were born, but it’s not the same as biological age, which is actually a predictor of their physiological health and life expectancy,” said Hugo Aerts, the study’s co-senior author, director of MGB’s Artificial Intelligence in Medicine program, and professor of radiation oncology at HMS. “A person’s biological age is dependent on many factors, like lifestyle, genetics, and other health factors. We had this idea that how old a person looks could actually be a reflection of their biological age.”
Led by scientists at MGB’s Artificial Intelligence in Medicine Program, the researchers trained FaceAge, their deep-learning algorithm, on more than 58,000 photos of healthy individuals of known age and on more than 6,000 photos of cancer patients whose age and clinical outcome was known.
The algorithm indicated that cancer patients’ FaceAge averaged five years older than their chronological age. It also found that looking older was associated with worse outcomes for patients suffering from several cancer types.
“We had this idea that how old a person looks could actually be a reflection of their biological age.”
Hugo Aerts
Judging one’s health according to appearance is nothing new, Aerts said. Doctors routinely make a visual assessment — the “eyeball test,” Aerts called it — when they walk in the room. It can encompass things like whether the patient is in a wheelchair, how robust they look, and whether they’re obviously ill.
The research showed, however, that the eyeball test — at least when performed by human physicians — is not a very good predictor of short-term life expectancy.
Published in the journal The Lancet Digital Health in early May, the study, which received funding from the National Institutes of Health, asked 10 clinicians and researchers to predict short-term life expectancy using photos of 100 terminal patients who were receiving palliative radiation therapy.
On average, they performed only slightly better than random chance, even when they knew things like the patient’s chronological age and the status of their cancer. Prediction improved, however, when the physicians were provided with FaceAge information for those patients.
Raymond Mak, a faculty member at the Artificial Intelligence in Medicine Program, HMS associate professor of radiology oncology, and co-senior author of the study, said having a better understanding of a patient’s biological age and how much time they likely have remaining allows oncologists to better tailor treatments.
He described a lung cancer patient who, though chronologically 86, looked considerably younger. That was a factor in Mak suggesting more aggressive treatment. Today, the man continues to do well at age 90. When Mak used FaceAge to analyze a photo of the patient at the time of treatment, the algorithm put his biological age as 10 years younger than his chronological age.
The opposite can also be true, Mak said, and patients who are frailer than their chronological age might suggest may need less-intensive treatment because that’s what their body can tolerate.
“We hypothesize that FaceAge could be used as a biomarker in cancer care to quantify a patient’s biological age and help a doctor make these tough decisions,” Mak said.
FaceAge has proved effective across several different types of cancer, Mak and Aert said, and they’re exploring its potential usefulness to predict outcomes in other diseases.
The algorithm employs deep learning, which means that it learns as researchers train it on thousands of photographs of people whose outcomes are known.
Researchers, however, don’t know which specific cues draw FaceAge’s focus, Aert said. It’s likely the algorithm is picking up on different things than a doctor might, such as wrinkles, gray hair, and baldness. If that’s true, that would make it particularly useful, he said, because it brings a different perspective to the physicians’ analysis of the patient’s condition.
Aerts and Mak said FaceAge would not be used on its own to determine courses of action but rather would be a tool available to physicians. It could not only help to determine initial treatment, but it could also monitor changes over time, alerting a doctor if a patient appears to be going downhill.
Before it is used in the clinic, however, it needs additional testing on diverse patient populations.
“In the clinic, the impact can be very large, because we now have a way to actually very easily monitor a patient’s health status continuously — before, during, and after treatment — and this could help us to better predict the risk of complications after, for example, a major surgery or other treatments,” Aerts said.