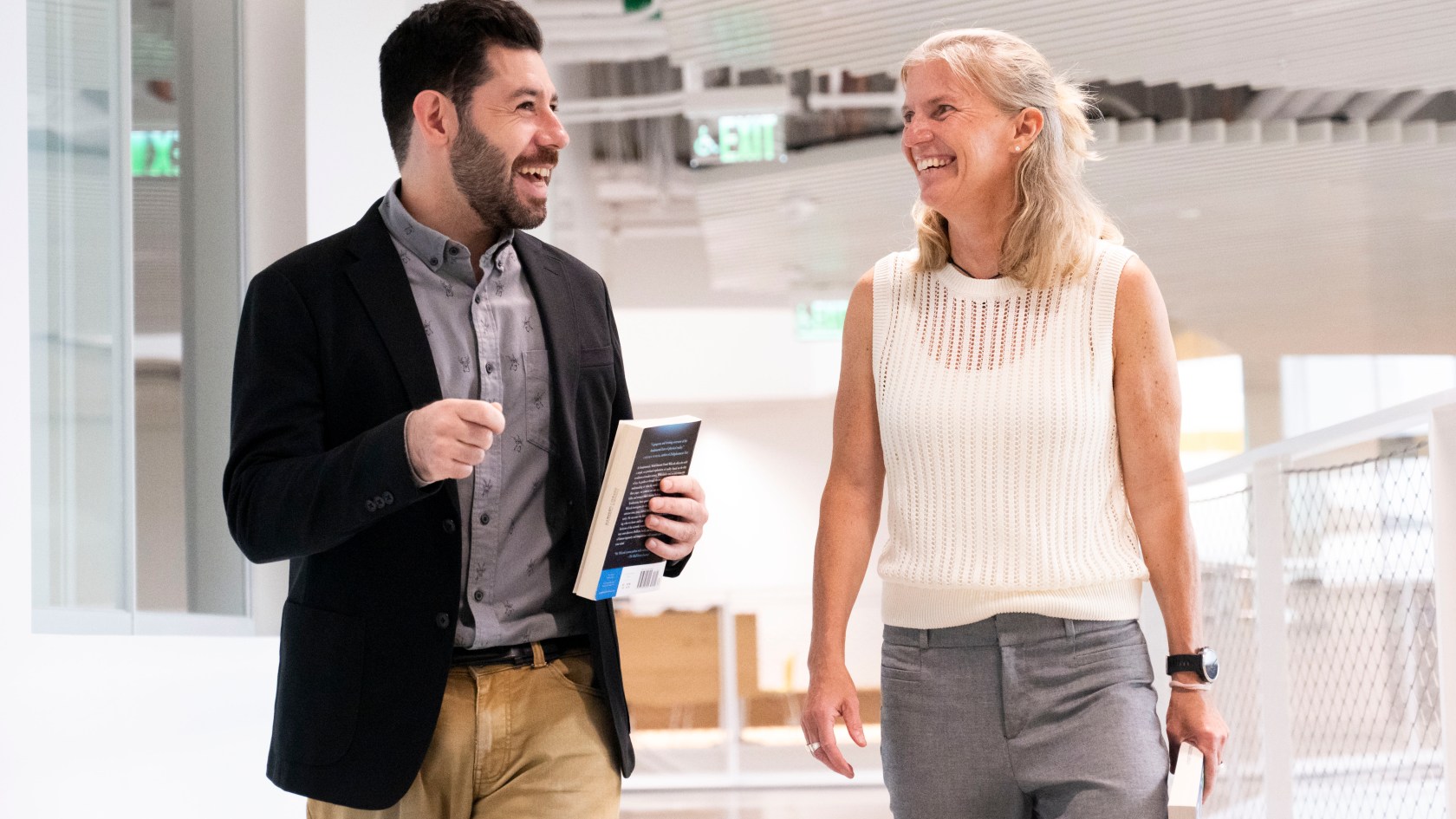
Study authors Gregory Michael Kestin and Kelly Miller.
Photo by Jon Ratner
Professor tailored AI tutor to physics course. Engagement doubled.
Preliminary findings inspire other large Harvard classes to test approach this fall
Think of a typical college physics course: brisk notetaking, homework struggles, studying for tough exams. Now imagine access to a tutor who answers questions at any hour, never tires, and never judges. Might you learn more? Maybe even twice as much?
That’s the unexpected takeaway from a Harvard study examining learning outcomes for students in a large, popular physics course who worked with a custom-designed artificial intelligence chatbot last fall. When compared with a more typical “active learning” classroom setting in which students learn as a group from a human instructor, the AI-supported version proved to be surprisingly more effective.
The study was led by lecturer Gregory Kestin and senior lecturer Kelly Miller, who analyzed learning outcomes of 194 students enrolled last fall in Kestin’s Physical Sciences 2 course, which is physics for life sciences majors. The final results are pending publication. Prior to the study, the team drew on their teaching and content expertise to craft instructions for the AI tutor to follow for each lesson so it would behave like a seasoned instructor.
“We went into the study extremely curious about whether our AI tutor could be as effective as in-person instructors,” Kestin, who also serves as associate director of science education, said. “And I certainly didn’t expect students to find the AI-powered lesson more engaging.”
But that’s exactly what happened: Not only did the AI tutor seem to help students learn more material, the students also self-reported significantly more engagement and motivation to learn when working with AI.
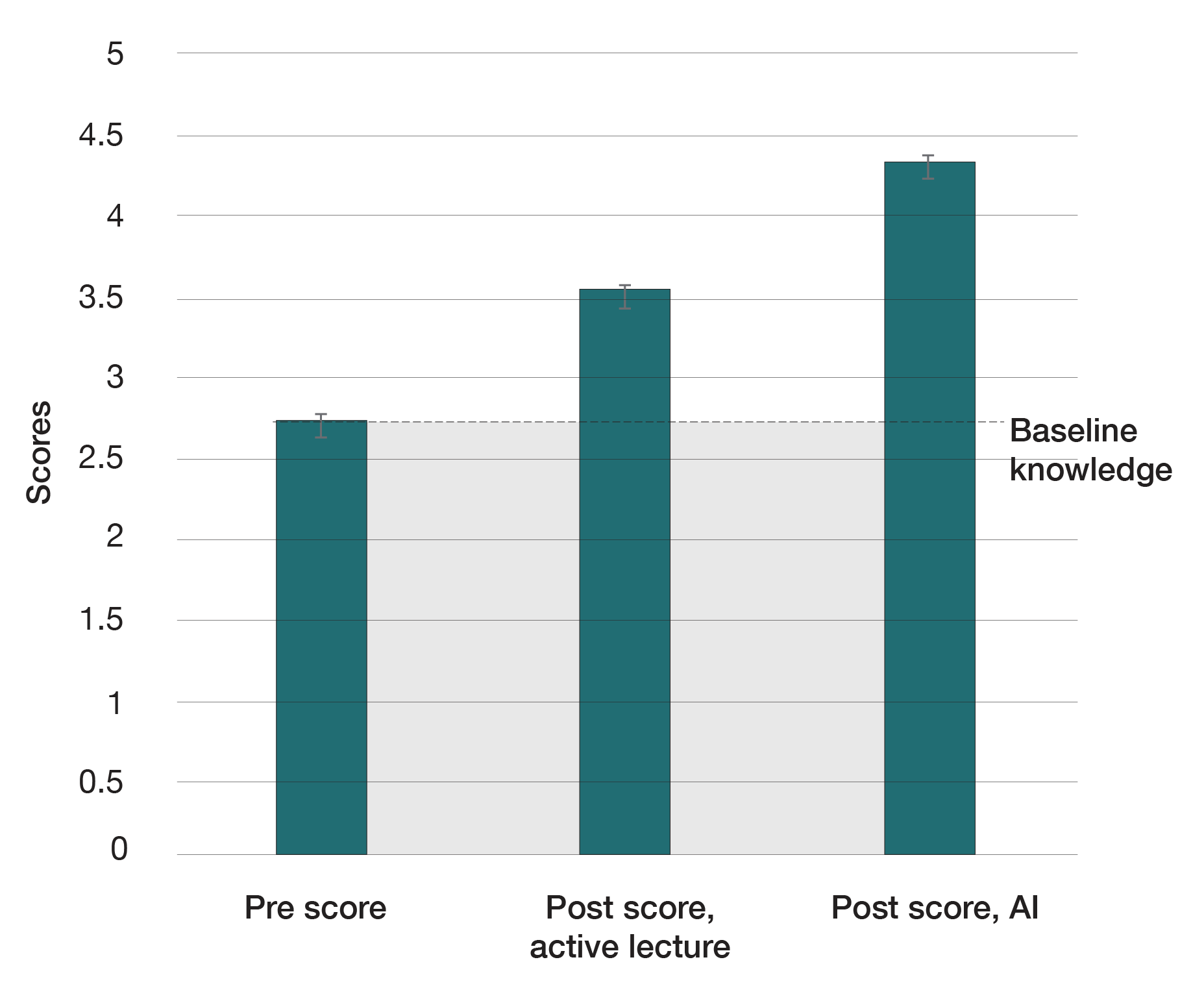
A comparison of mean post-test performance between students taught with the active lecture and students taught with the AI tutor. Dotted line represents students’ mean baseline knowledge before the lesson (i.e. the pre-test scores of both groups).
Source: “AI Tutoring Outperforms Active Learning,” Gregory Kestin, Kelly Miller, Anna Klales, Timothy Milbourne, Gregorio Ponti
“It was shocking, and super exciting,” Miller said, considering that PS2 is already “very, very well taught.”
“They’ve been doing this for a long time, and there have been many iterations of this specific research-based pedagogy. It’s a very tight operation,” Miller added.
The experiment shows the advantage of using AI tutoring as students’ first substantial introduction to challenging material, the researchers wrote in their paper. If AI can be used to effectively teach introductory material to students outside of class, this would allow “precious class time” to be spent developing “higher-order skills, such as advanced problem-solving, project-based learning, and group work,” they continued.
Though excited by AI’s potential to revolutionize education, Kestin and Miller are cognizant of potential misuses.
“While AI has the potential to supercharge learning, it could also undermine learning if we’re not careful,” Kestin said. “AI tutors shouldn’t ‘think’ for students, but rather help them build critical thinking skills. AI tutors shouldn’t replace in-person instruction, but help all students better prepare for it — and possibly in a more engaging way than ever before.”
The Institutional Review Board-approved study took place in fall 2023. Nearly 200 students consented to be enrolled in the study, which involved two groups, each of whom experienced two lessons in consecutive weeks. During the first week, Group 1 participated in an instructor-guided active learning classroom lesson, while Group 2 engaged with an AI-supported lesson at home that followed a parallel, research-informed design; conditions were reversed the following week.
The researchers believe that students’ ability to get personalized feedback and self-pace with the AI tutor are advantages compared with in-class learning.
The study authors compared learning gains from each type of lesson using pretests and posttests to measure content mastery. They also asked the students how engaged they felt with each type of instruction, how much they enjoyed each type, how motivated they were, and how they would assess their “growth mindset.”
Learning gains for students in the AI-tutored group were about double those for students in the in-class group, according to the preliminary study analysis. The researchers believe that students’ ability to get personalized feedback and self-pace with the AI tutor are advantages compared with in-class learning.
In various in-class settings, “Students who have a very strong background in the material may be less engaged, and they’re sometimes bored,” Miller said. “And students who don’t have the background sometimes struggle to keep up. So the fact that this [AI tutor] can be supportive of that difference is probably the biggest thing.” This is especially valuable when students are first being introduced to concepts and problems on topics that only some students have seen before, the researchers said.
Miller stressed that the AI tutor was customized with research-based prompt engineering and “scaffolding” to ensure the lessons were accurate and well-structured.
Kestin began creating the website that hosts the PS2 tutor the previous summer, shortly after ChatGPT made its global debut. The framework is built on the GPT application programming interface, and it is structured so that conversations, including the AI tutor’s personality and quality of feedback, are pre-vetted. So rather than defaulting to ChatGPT behavior, the custom tutor provides users with information guided by content-rich prompts that have been refined and placed into the framework.
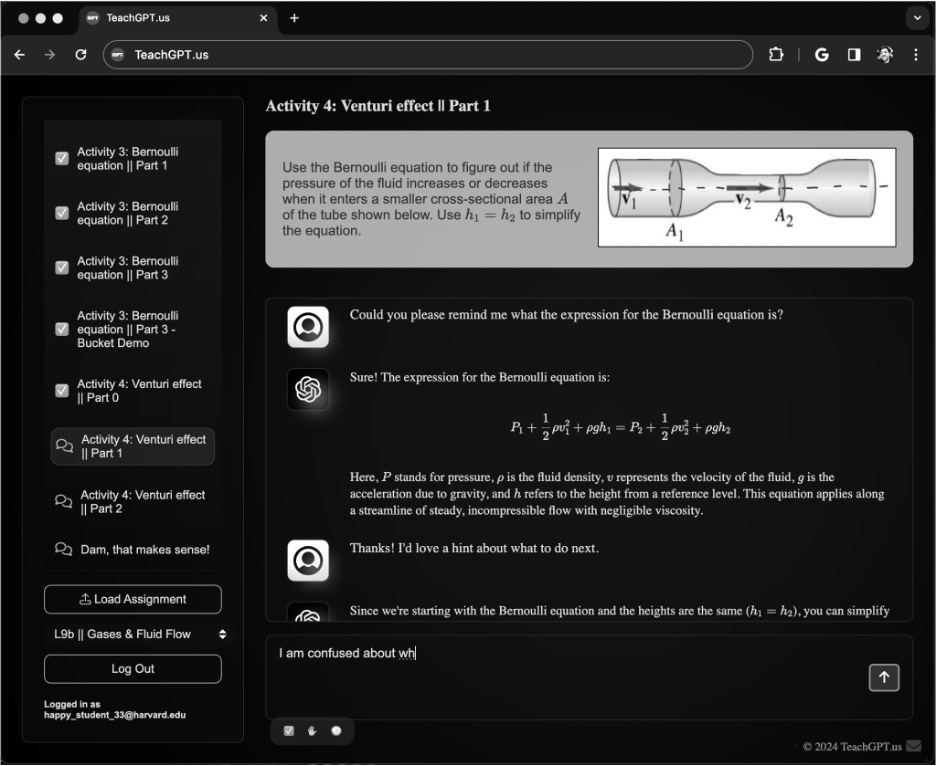
Once the framework was built, it was easy to start customizing it for other courses and subject matters, Kestin said, which is why several colleagues are already trying it out.
Mathematics instructor Eva Politou will introduce a version of Kestin’s AI tutor to Math 21a (Multivariable Calculus) this fall in the workshop portion of the course, which is typically taught by undergraduate course assistants. Every week, students will be able to generate questions about a specific topic and search for answers with the AI tutor acting as a guide.
“The primary goal of the AI tutor is to promote an inquiry-based studying method,” Politou explained. “We want students to practice generating questions, critically approaching real-life scenarios, and becoming active agents of their own understanding and learning.”
Inspired by Kestin and Miller’s results, the Derek Bok Center for Teaching and Learning is collaborating with Harvard University Information Technology to pilot similar AI chatbots in a handful of large introductory courses this fall. They are also developing resources to enable any instructor to integrate tutor bots into their courses.
The study was co-authored by Anna Klales, Timothy Milbourne (PS2 co-instructor), and Gregorio Ponti, all of whom teach in the Department of Physics.