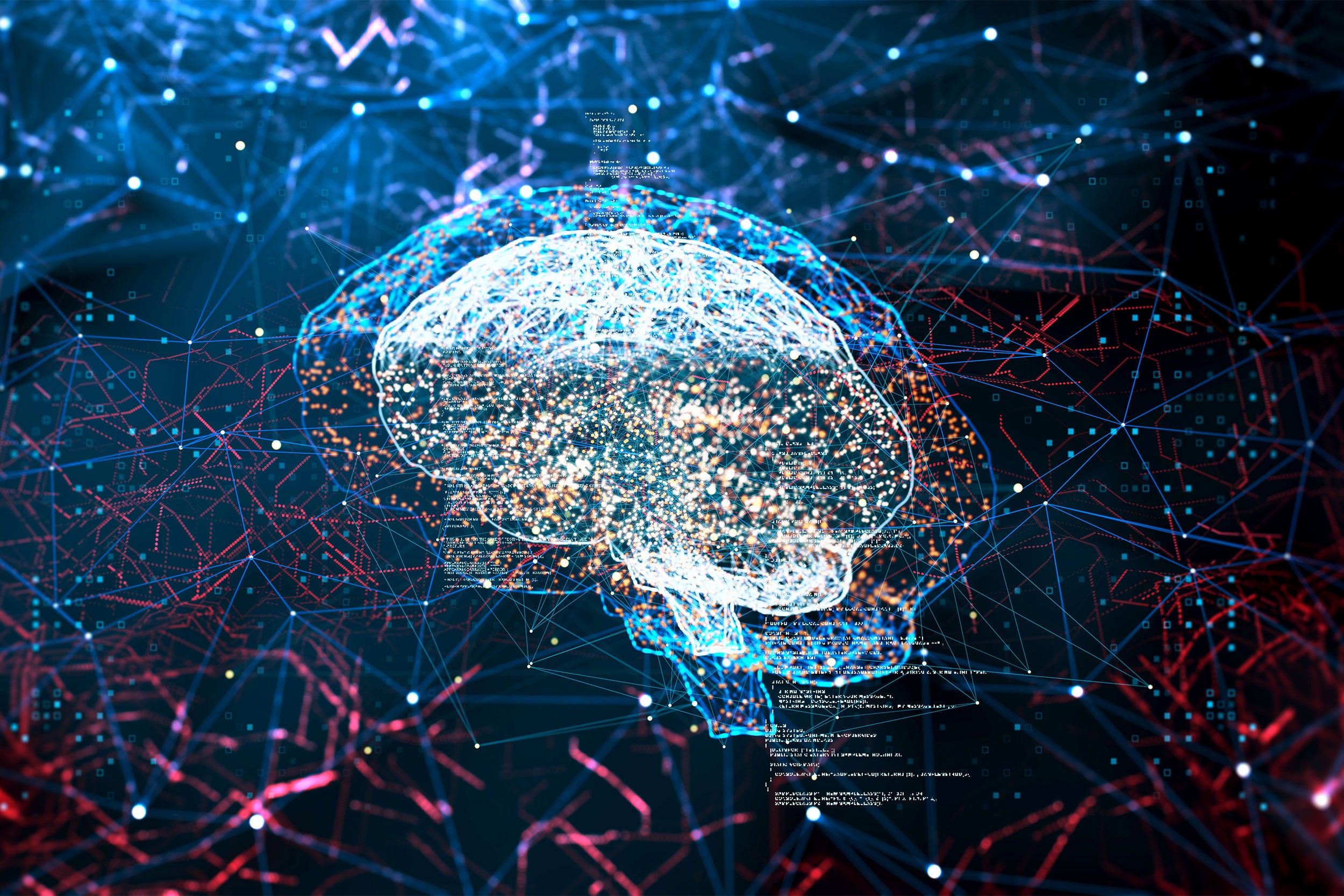
Aramont Fellows bring cutting-edge scientific innovation to the forefront
Four groundbreaking projects investigate brain development, capture raw data with AI, innovate quantum computers, and develop new models to map supernovas
Since 2018, the Aramont Fellowships have recognized cutting-edge scientific innovation. It is notoriously difficult for early career researchers to receive funding for high-risk projects, and many scientists often miss out on valuable opportunities to further their research. Enter the Aramont Charitable Foundation, which presents the Aramont Fellowship Fund for Emerging Science Research Awards to a carefully selected group of postdocs and early-career faculty whose work shows tremendous promise. This year’s recipients are tackling varied topics in their funded projects, from quantum computing to investigating protein degradation in the brain.
“I continue to be inspired by the caliber of our applicant pool for the Aramont Fellowship program, and I am very excited about this cohort of awardees,” says Vice Provost for Research John Shaw. “These investigators have proposed projects that will expand the boundaries of their fields, and we have much to learn from the work being funded.”
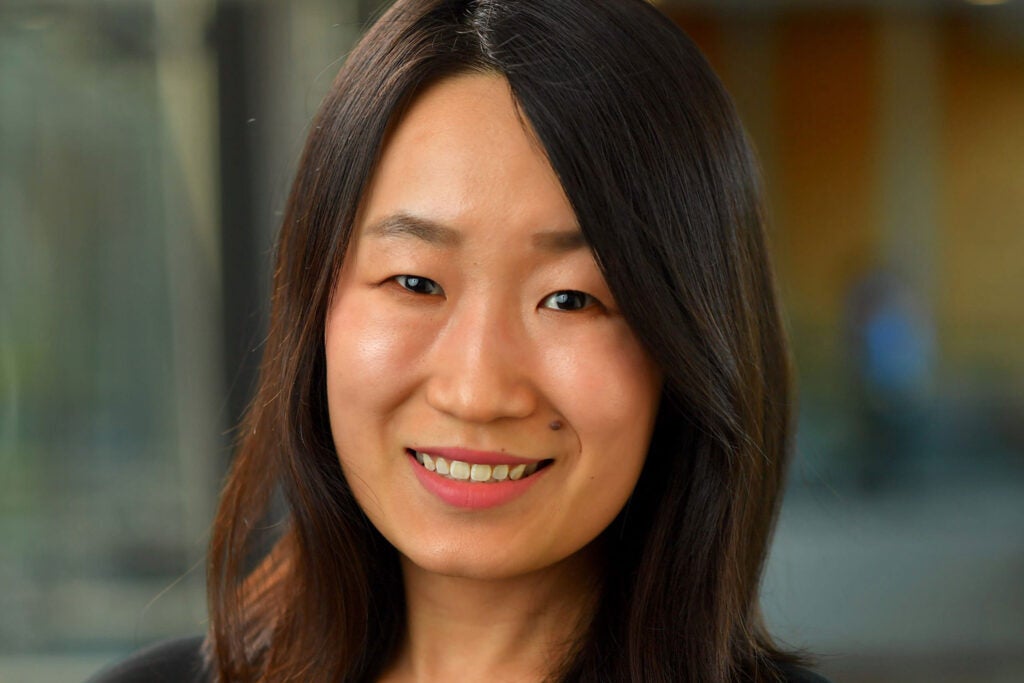
Postdoctoral Fellow in Neurobiology, Harvard Medical School
“Modulating Brain Functions via a Novel Protein Degradation Pathway”
Sensory stimuli that activate brain development and adaptability induce the expression of a set of genes called immediate early genes (IEGs). While essential for brain function, the mechanisms that control the expression of IEG proteins have remained elusive for more than forty years. Gu discovered a stimuli-induced protein called midnolin that is believed to have broad functions in neuronal physiology. This discovery may inspire many new research directions, including exploring how midnolin functions mechanistically. Gu’s work also found that a complex involving midnolin is attracted to chromatin—a key component of human and animal chromosomes. She will explore midnolin’s effects on chromatin, as well as the physiological functions of midnolin, for which she has generated a mouse model that will be used to investigate the role of midnolin in learning and memory.
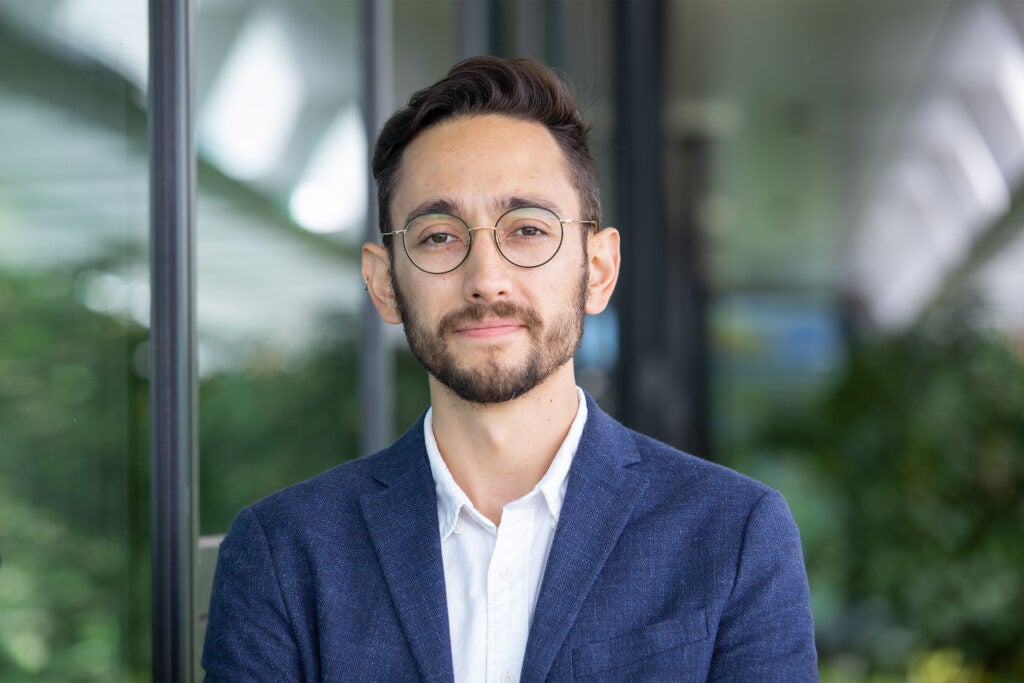
David Alvarez-Melis
Assistant Professor of Computer Science, Harvard John A. Paulson School for Engineering and Applied Sciences
Photo by Eliza Grinnell
“Accelerating Scientific Research with Large Multi-Modal AI Models”
Modern scientific discovery is driven by insights drawn from large amounts of complex, multiformat, experimental data, and processing and analyzing this data is a laborious process that could potentially be facilitated by AI. Large Language Models (LLMs), with their impressive knowledge aggregation and retrieval capabilities, are particularly appealing for this task. However, the scientific knowledge of current LLMs is outdated and unalterable and based mostly on text sources. Melis’ lab believes this is unlikely to be sufficient to fuel the next scientific breakthrough. Instead, novel discoveries will most likely be made from experimental data still being analyzed or perhaps still being generated in an observatory, a wet lab, or a particle accelerator. Letting LLMs tap into that trove of data could help achieve breakthroughs, but would require rethinking their form, function, and use — specifically, to leverage the “reasoning” abilities of LLMs while enhancing them with the capacity to ingest and process large amounts of “fresh” multiformat data like images, graphs, or tables, so that LLMs can ultimately spot patterns and suggest future experiments to radically accelerate the pace of scientific research.
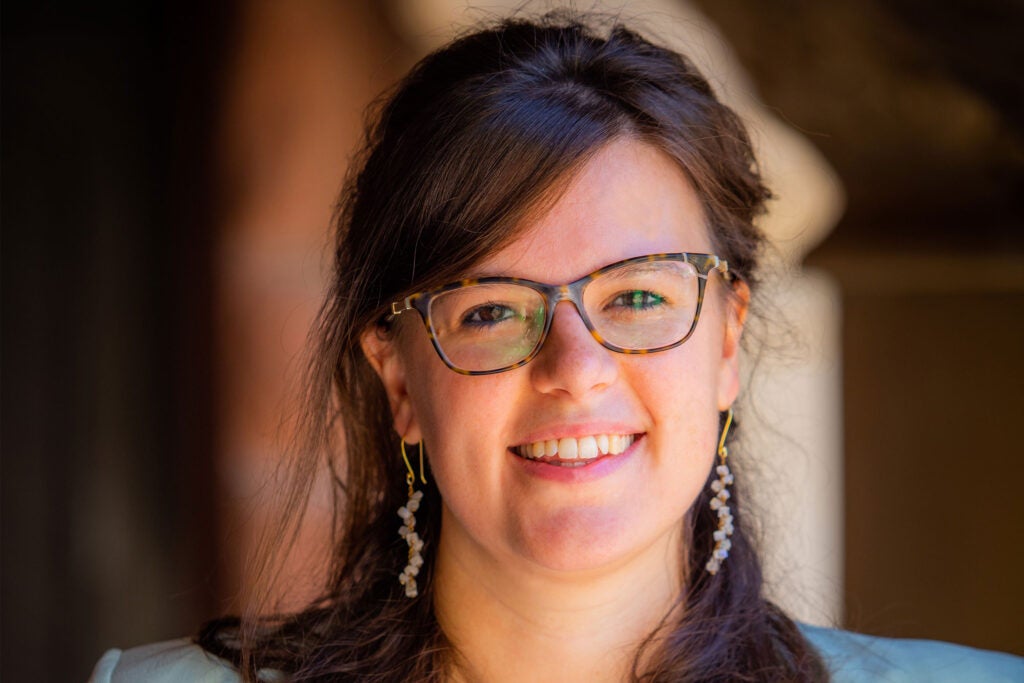
Assistant Professor of Applied Physics, Harvard John A. Paulson School for Engineering and Applied Sciences
“Advancing Programmable Quantum Computers: Overcoming Challenges and Expanding Capabilities with Dual-species Atom Arrays”
Quantum computers promise to solve problems that even the most powerful supercomputers cannot by leveraging fundamental principles of quantum mechanics. The impressive progress of this platform over the last few years has brought the field to an important juncture, and it is imperative for the field to address pressing challenges related to scalability, resistance to errors, and flexibility.
Semeghini seeks to introduce innovative capabilities to programmable atom arrays, extending the platform’s scope and addressing current limitations by combining two atomic species with complementary features within the same array. This will enable new approaches to error correction and scalability, which are crucial for the development of quantum architectures pushing the boundaries of quantum computers, and for enabling these computers to eventually solve real-world problems. This work will also extend the range of applications to a broad variety of questions related to information processing and optimization as well as fundamental questions in condensed matter, high-energy physics, and quantum chemistry.
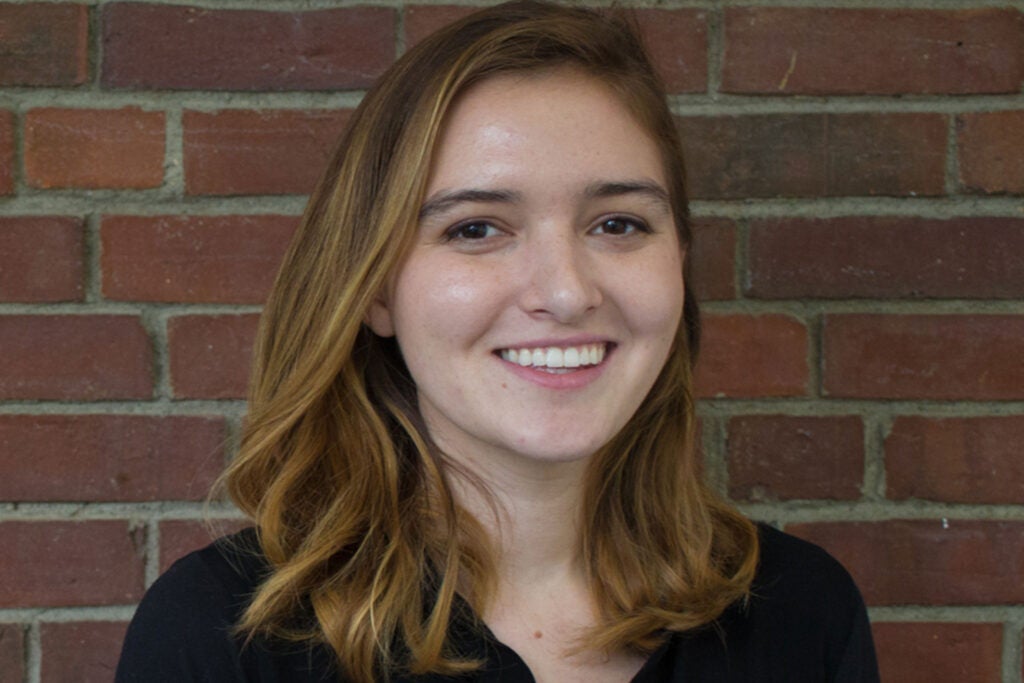
Assistant Professor of Astronomy, Faculty of Arts and Sciences
“A Foundation Model for Time-domain Astrophysics”
Studying the explosive deaths of stars enables astronomers to understand high-energy physics, test evolutionary models of stars, and measure the expansion of the universe itself. Currently, around 10,000 explosive cosmic events are discovered annually, and the pace of discovery will only speed up as more advanced technologies come online in the next few years. Researchers need new techniques to efficiently classify these events and identify novel high-energy physics. Villar’s project will integrate broadband light curves, high-fidelity spectroscopy, and images into a unified model for real-time analysis of our variable night sky. Her work aims to produce the first astrophysical time-domain foundation model, an algorithm using AI and raw data to perform a variety of tasks, which will then be used to classify new events, identify novel physics, and rapidly infer physical parameters for known phenomena in real time.