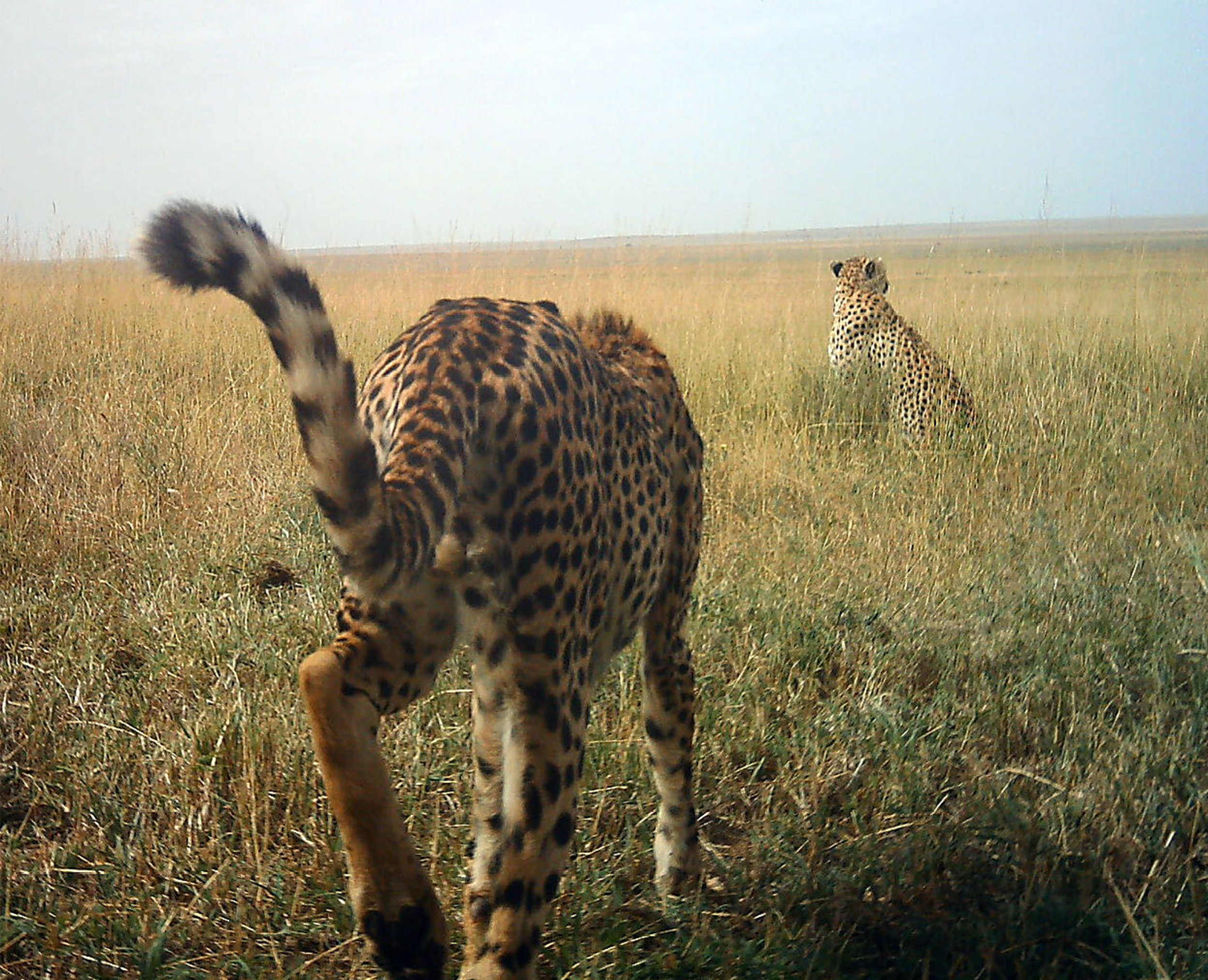
Artificial intelligence correctly identifies two cheetahs in this image captured with a motion-sensor camera in Tanzania.
Photos courtesy of Snapshot Serengeti
“Deep learning,” already poised to transform fields from earthquake prediction to cancer detection to self-driving cars, is about to be unleashed on a new discipline — ecology.
A team of researchers from Harvard, Auburn University, the University of Wyoming, the University of Oxford, and the University of Minnesota has demonstrated that the artificial-intelligence technique can be used to identify animal images captured by motion-sensing cameras.
Researchers applied deep learning to more than 3 million photographs from the citizen-science project Snapshot Serengeti to identify, count, and describe animals in their natural habitats. The system was able to automate the process for up to 99.3 percent of images as accurately as human volunteers. The study was described in a paper published last month in the Proceedings of the National Academy of Sciences.
Motion-sensitive cameras deployed in Tanzania by Snapshot Serengeti collect images of lions, leopards, cheetahs, elephants, and other animals. While the images can offer insight into a range of questions, from how carnivore species coexist to predator-prey dynamics, they are only useful once they have been converted into data that can be processed. For years, the best method for extracting such information was to ask crowdsourced teams of volunteers to label each image manually.
“Not only does the artificial intelligence system tell you which of 48 different species of animal is present, it also tells you how many there are and what they are doing,” said Harvard’s Margaret Kosmala, one of the leaders of Snapshot Serengeti and a co-author of the study. “It will tell you if they are eating, sleeping, if babies are present, etc.
“We estimate that the deep-learning technology pipeline we describe would save more than eight years of human labeling effort for each additional 3 million images. That is a lot of valuable volunteer time that can be redeployed to help other projects.”
“We estimate that the deep-learning technology pipeline we describe would save more than eight years of human labeling effort for each additional 3 million images.”
Margaret Kosmala
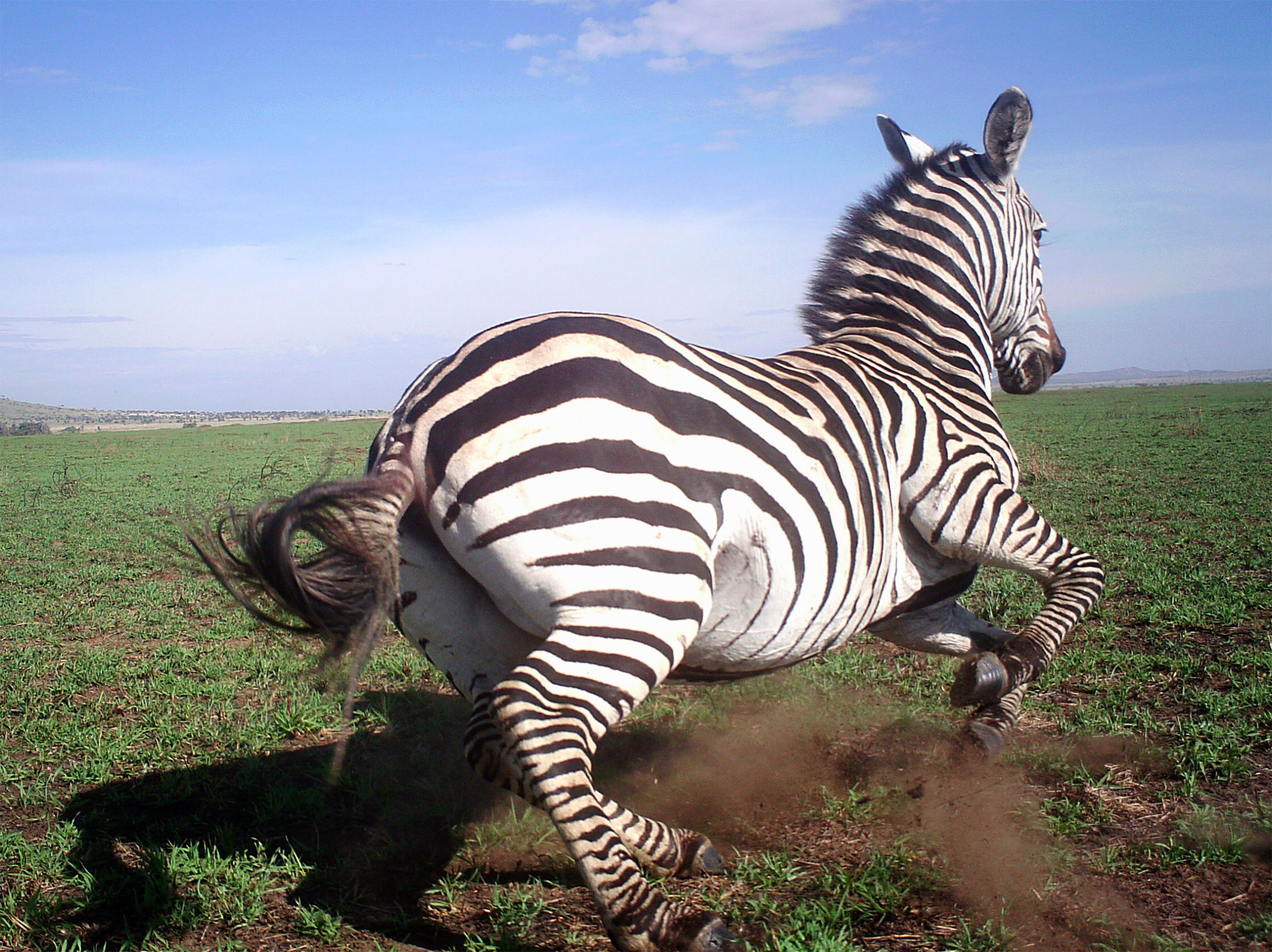
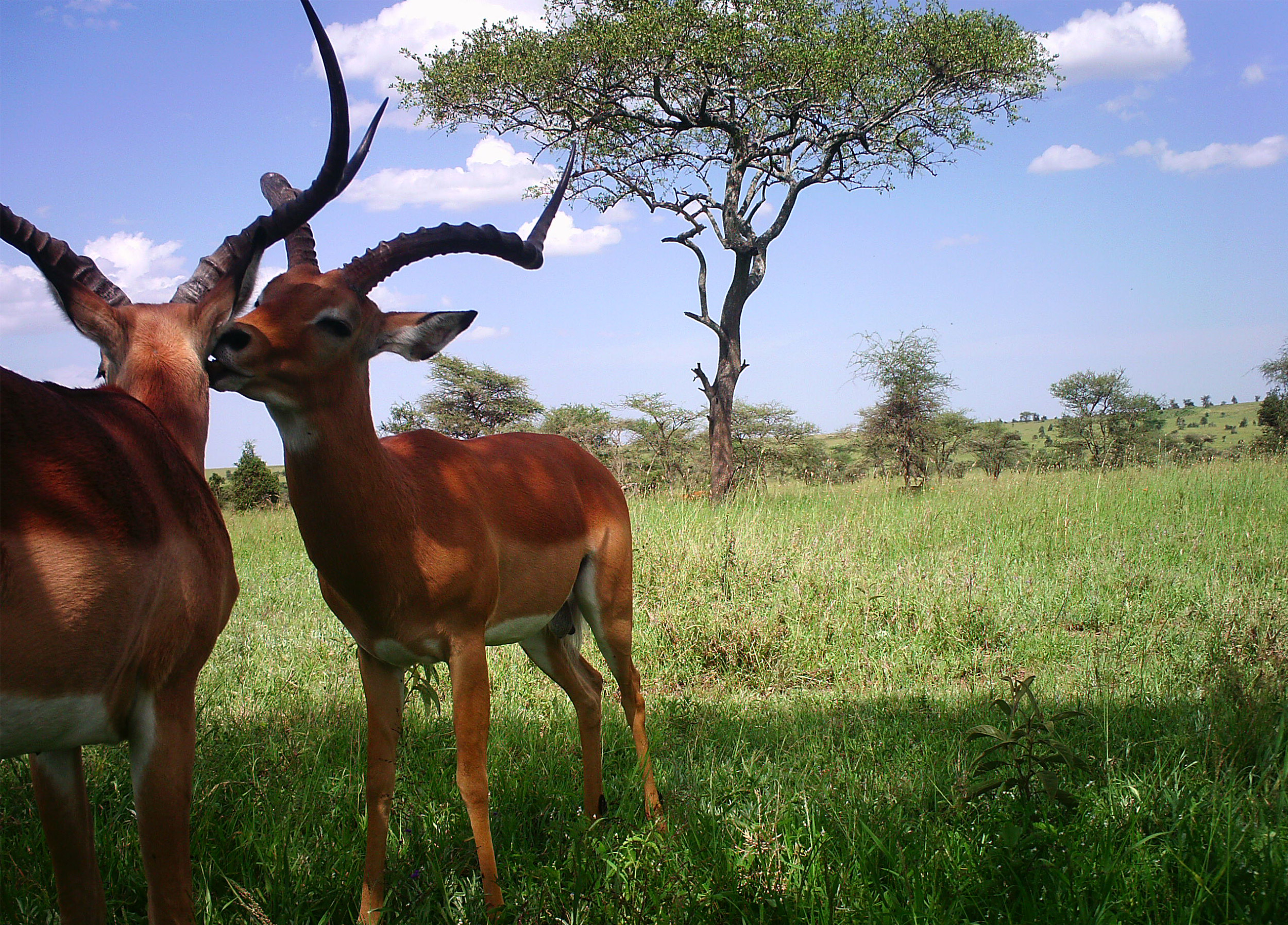
The AI system labels and counts the animals pictured and describes what they are doing. For the images above it reports “a zebra moving” and “two impala standing.”
“While there are a number of projects that rely on images captured by camera traps to understand the natural world, few are able to recruit the large numbers of volunteers needed to extract useful data,” said Snapshot Serengeti founder Ali Swanson. “The result is that potentially important knowledge remains locked away, out of the reach of scientists.
“Although projects are increasingly turning to citizen science for image classification, we’re starting to see it take longer and longer to label each batch of images as the demand for volunteers grows,” Swanson added. “We believe deep learning will be key in alleviating the bottleneck for camera-trap projects: the effort of converting images into usable data.”
“Our citizen scientists have done phenomenal work, but we needed to speed up the process to handle ever greater amounts of data,” said Craig Packer, who heads Snapshot Serengeti. “The deep-learning algorithm is amazing and far surpassed my expectations. This is a game changer for wildlife ecology.”
First author Mohammad Sadegh Norouzzadeh expects that deep-learning algorithms will continue to improve, and hopes to see similar systems applied to other ecological data sets.
“Here, we wanted to demonstrate the value of the technology to the wildlife-ecology community, but we expect that as more people research how to improve deep learning for this application and publish their data sets, the sky’s the limit,” he said. “It is exciting to think of all the different ways this technology can help with our important scientific and conservation missions.”
This research was supported with funding from the National Science Foundation.