
“Most important is to create opportunities for scientists here to interact in new ways,” said Francesca Dominici, co-director of the Harvard Data Science Initiative with David C. Parkes (not pictured), George F. Colony Professor and area dean for computer science at SEAS.
Kris Snibbe/Harvard Staff Photographer
Data science for a new era
A Q&A with co-directors of emerging Data Science Initiative
Harvard University just announced the launch of its Data Science Initiative, a program to harness the vast expertise and innovations that are occurring in disciplines as diverse as medicine, law, policy, and computer science.
Initiative co-directors Francesca Dominici, professor of biostatistics at the Harvard T.H. Chan School of Public Health, and David C. Parkes, George F. Colony Professor and area dean for computer science at the Harvard John A. Paulson School of Engineering and Applied Sciences, are enthusiastic about the work ahead.
In a Q&A session, Dominici and Parkes talked with The Gazette about their vision for the initiative and how data science can address serious challenges that confront individuals and society.
More like this
GAZETTE: The term “data science” gets thrown around a lot, but what is it?
PARKES: You get different definitions from different people. It is the “science of data.” It is doing science through data: How can we glean impactful new knowledge from data? It is scaling up computation: How do we design efficient algorithms for very large data sets? How do we visualize data? Those types of things.
DOMINICI: I think what’s most exciting about data science is that we are developing new methodologies or tailoring existing methodologies in the context of applications that matter — in other words, not having scientists work in the abstract. If they’re developing a new methodology, they’re doing it because there’s a particular challenge, an application of data science that is driving them. And that’s a wonderful opportunity if we can get that happening in the right way.
GAZETTE: Why is the time right for a data science initiative at Harvard?
PARKES: It’s a confluence of three things. One is that we have a lot more data than we’ve ever had. But it’s not just more data, it’s different kinds of data as well. Harvard researchers are eager to use this data to make research breakthroughs. The second thing is that we have very mature computational platforms now, including things that we wouldn’t have anticipated using for data science. For example, a lot of the computation is happening on computers that had been developed for video games. You may have heard of GPUs, graphical processing units. GPUs can process large amounts of high-dimensional data in a very parallel way. Third, new algorithms are being developed. It’s really all coming together at the same time.
GAZETTE: We’re talking about data, but what exactly does that mean? What kinds of information are people using? How much of it is there? And how are scientists using it?
DOMINICI: Because of the new advances in technology, almost every field right now has data, and more data than ever. Clearly, there’s the explosion of genetics and genomics data in the life sciences, in molecular data, as well as astronomy and economics. Even in the humanities, you can scan documents and turn it into data that you can analyze. If you think about it, even ourselves, with our smart mobile devices, we have more data than ever before. So, there is data everywhere. Sometimes it’s big and massive. Sometimes it’s not big, but it’s complex and it’s in different formats. We have the opportunity to glean knowledge from this data.
PARKES: To add some numbers to this, IBM has estimated that we’re generating more than one quintillion bytes of data a day. (A quintillion is a 10 to the 18th.) In other words, the data all of us have generated, however they measure it, over the past two years is as much as 90 percent of the data that’s ever been generated. Now, this may not be the kind of data that we normally think about in academic work. It’s the data that’s harvested all the time from our everyday activities. But it includes the very-high-volume data that Francesca was referring to in the medical space as well. The point she alluded to is really important, which is having more data is a very good and very helpful thing because you can get more statistically significant signals from your data. You can understand the true pattern there without being overwhelmed by noise.
But there are challenges. You’ve got to push all that data around. You’ve got to store it. You’ve got to scalably compute on it.
“Bringing together such an unusually broad range of expertise is how we will use data science to tackle some of the world’s most vexing problems.” — Francesca Dominici
GAZETTE: What are some of the challenges or problems that data science can help us address? What are some real-world, tangible applications?
PARKES: Here’s one possibility: What if we could make machine reading and machine understanding get to the point where we can ingest all of the scientific literature and all of the science journalism out there and actually create an algorithm that can instantly ingest new findings and update some kind of “knowledge base” that could be used to improve decision-making? At the moment, there’s too much literature for anyone to possibly keep up. There have been many advances in natural language understanding recently, using statistical machine learning. What if we could actually harness this to understand the medical literature and keep on top of it? This is something that people are trying to work on.
DOMINICI: One of the reasons we are so excited that Harvard is launching the Data Science Initiative is because of all the advances our faculty have made in recent years. We can now describe the entire genome, define the exposome (the environmental analogue to the genome), characterize social interactions and mood via cellphone data, and can digitize historical data relevant for the humanities.
Let me give just a couple more examples. One is in the context of climate change and environmental policy, my own work, and the second in the context of personalized medicine. We are developing statistical models, algorithms, and scalable tools to estimate the health effects of air pollution and the effectiveness of EPA [Environmental Protection Agency] air quality regulations. Data science can yield evidence to support cost-effective regulations and form the basis of sound policy.
A second example is using genomics data and electronic medical records to create personalized treatment strategies, to help clinicians take patients’ individual characteristics into account for treatment, to potentially revolutionize how we approach patient care and enable precision medicine.
PARKES: Returning to the social sciences, pioneering work by Harvard researchers is using new kinds of data — specifically street-view images of urban landscapes — to understand connections between urban appearance and how people live in cities, including questions about urban growth, income, and crime. Data science provides tools with which to understand the causal effect of policy interventions, such as a new greenway or light-rail system, on urban change. This research exemplifies the use of crowdsourcing in curating new data sets, with deep neural nets and methods from computer vision used to build predictive models at a scale and fidelity that would have been hard to imagine just a few years ago.
GAZETTE: What do you think that Harvard brings to the field of data science, or that this initiative will bring to the field, that isn’t already there?
DOMINICI: First of all, scale. We have a colossal number of faculty working with data across campus who are eager to push forward the field of data science not only for research but also for education. The breadth and scope of expertise here at Harvard is astounding. We have data science experts in our Schools of medicine, public health, business, law, arts and sciences, government, education, and engineering, all of whom are actively engaged in this field. This new initiative will provide the structure to bring them together, to amplify and augment the power of their work. Bringing together such an unusually broad range of expertise is how we will use data science to tackle some of the world’s most vexing problems. We are also seeing an enormous increase in interest from the students.
PARKES: Just in terms of the thirst for learning, I’m co-teaching our machine-learning class in computer science at the moment. We have more than 200 students in the class. There’s clearly a lot of built-up interest from our undergraduates to learn about various aspects of data science. We also have a new data science curriculum that we’ve launched, together with statistics, in response to this. We are building out education at the master’s level. This is happening in the Chan School of Public Health, in the Medical School, and in the Faculty of Arts and Sciences.
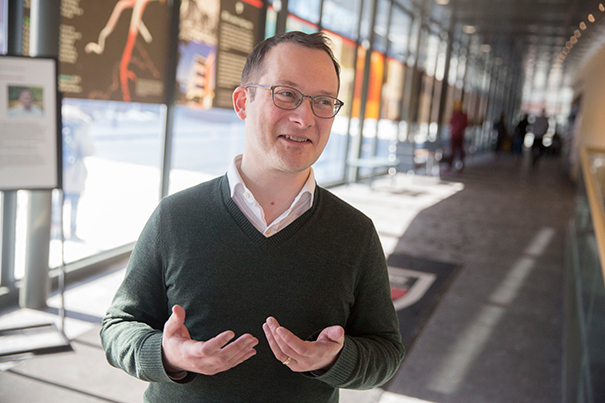
We also think that it’s imperative for Harvard to be advancing data science in a way where we can provide knowledge and methodologies to other researchers, and to the public and policymakers, as to what is the right way to answer particular kinds of questions. That seems to be an advantage that we have in our visibility. It means that we should be doing something here.
GAZETTE: What are your next steps? And where do you hope to be five or 10 years?
PARKES: Most important is to create opportunities for scientists here to interact in new ways. We will succeed if we can get people who are working on data-science-related topics all across the University to get to know each other better. And hopefully this will lead to new opportunities that they didn’t know existed. That sounds very mundane, but it’s hugely important. We can do this, for example, through running network events, half-day workshops, social events, and larger symposia.
GAZETTE: What are some elements that are already in place?
DOMINICI: We have launched the Harvard Data Science Postdoctoral Fellowship, which is among the largest programs of its kind, and we want to recruit talented individuals in a highly interdisciplinary ways. We’re looking for people who can lead their own research but will want to work collaboratively with other people around the University. In fact, we’ve asked them to identify faculty they’d be excited to work with. In addition to passion for computer science and statistics, we are are looking for talented individuals who want to advance knowledge in astronomy, psychology, business, health, and are excited to work with us to build data science at Harvard. We have a committee that will be making decisions about this very soon.
We have also launched a competitive research fund that will catalyze small research projects around the University. Through our friends in the Faculty of Arts and Sciences and the Medical School, we’ve identified some spaces in the near term where people can get together. For example, the postdocs would be able to make use of the space. We would be able to run networking events in the spaces. Other postdocs, other researchers could use the space. There’ll be space in the Science Center and space in one of the libraries over near the Medical School.
GAZETTE: What do you hope to see from your colleagues across the University? How do you want them to engage with this? How can they engage with it?
DOMINICI: There are 55 faculty now across all Schools of Harvard who are already really engaged as part of our governance structure. And we’re going to engage more. This is going to be a faculty initiative.
PARKES: We really want this to be organic. We want to be doing things that are useful for researchers across Harvard. We will be reaching out to them and networking. We’ve already been doing some of this, and we’ll keep doing it.
DOMINICI: I do think that because of where the science is, and because the incentive for faculty at Harvard to work together in the context of data science is very big right now, this is the right moment for this initiative. New collaborations will come that will have a big impact. I think some silos will be broken. Even cultivating the new generation of researchers who are seeing Harvard as an organic and integrative place for learning about how to analyze data, this could have an enormous impact. A lot can be done because the time is right and because there is the support of the University.
GAZETTE: Are there long-term plans?
PARKES: We are launching the initiative because we want to get to a point where we have a Harvard Data Science Institute. The aspiration is that the Data Science Institute will have some physical space associated with it, will provide resources to help with hiring new faculty around the University in the area of data science, and will, as we already said, be a kind of home in a programmatic way to support data science as well as a new cohort of professional, research data scientists.
GAZETTE: Some people have concerns about the potential misuse of big data. What are your thoughts on that?
PARKES: There are different kinds of concerns. One concern is making sure that we are building models that are transparent and well validated, and therefore that users can understand them. A model, as you might know, is a mathematical description of data, and we should ensure that our models are succinct, accessible, and understandable.
The second thing people worry about is fairness, and quite rightfully so. We don’t want models that reflect human biases. You need definitions of fairness, and then you need to encode those concepts in methods.
Then the third one I wanted to mention is privacy. One example of progress in this area is research by my colleague Cynthia Dwork, who’s a new faculty member here at Harvard. She, with her colleagues, introduced the idea of defining something called “differential privacy.” What differential privacy means is that if I change any data about one individual, it shouldn’t change the conclusions of the data analysis by very much. In other words, the outputs are not sensitive to one user’s data being there or not. Because of that, you cannot infer anything about that one individual’s data. So again, this gets to a very important societal concern. It’s been recognized as such by scientists, legal scholars, statisticians, and computer scientists. As scientists, as researchers, we should all care about this and make sure that we’re doing the right thing. It’s very important.