It’s the missing data, stupid!
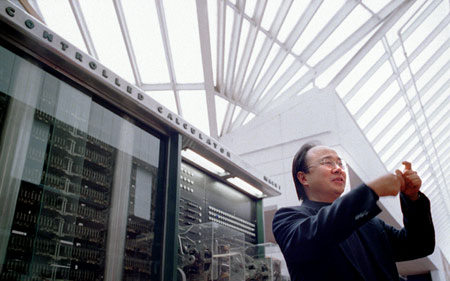
Xiao-Li Meng is a bit different from other scientists. He not only works with the data he has, he works with the data he doesn’t have.
Meng, appointed a professor of statistics last July, is one of the world’s foremost authorities on missing data, something that scientists crave, and something that Meng helps them understand and deal with.
While Meng cannot produce the missing data itself, he uses statistical methods on an array of real-world problems to help researchers understand enough about the data to get an idea of why it’s missing and whether and how it affects their analyses and conclusions.
As science grows more complex, the importance of what scientists don’t know grows larger and larger. In everything from public polling to genetic research to questions of global warming, the missing information is important, perhaps critically important.
Statistics Department Chair Donald Rubin said Meng’s enthusiasm and active mind have made him well known in the statistics community. Meng won the 2001 COPSS Award, from the Committee of Presidents of Statistical Societies, the most prestigious award granted annually to a single statistician under the age of 40. He is known for work in several different areas, including finding new, more general uses for pre-existing statistical theories.
“He’s just extremely creative in that kind of area and technically very strong,” Rubin said. “He’s also an enthusiastic and generous teacher, generous with his time and ideas both with advanced and beginning students.”
Meng, teaching two undergraduate classes this semester, said he enjoys teaching and advising “immensely.” He won a University of Chicago faculty award for excellence in graduate teaching and has already been invited to student-faculty dinners at undergraduate houses by a number of students as their favorite professor.
“I have always been fascinated by the fact that my teachers were able to turn me from a completely ignorant little boy to who I am today, and it thrills me every time I realize that now I can be a part of another human being’s fascination,” Meng said.
In addition to his work on missing data, Meng is also known for contributions to statistical computing and computer simulations and to mathematical statistics in complex problems involving p-values, a measure of the significance of a hypothesis test. Meng is also known for the study of noninformative prior distributions, which is a statistical way of quantifying a lack of information.
Relative information and family trees
One of Meng’s current projects involves statistical genetics. He is working in collaboration with several researchers who analyze genetic data from Iceland, a nation that has made a national obsession of keeping meticulous family trees. As scientists on the project analyze genetic data, they have to figure out if particular traits are linked to particular genes. Part of the problem in their analysis is knowing when to stop. At a certain point, they will have extracted most of the useful information out of a genetic sample and it would be expensive to continue to process and analyze that sample.
The problem is that without knowing how much information they can squeeze from their incomplete data, they don’t know when they ought to call it quits and go get another sample – an expensive proposition in itself.
“Should I beat my current data to death, so to speak, or should I go collect new data?” Meng sums up the problem.
Meng said the problem may appear to be hopeless, but with certain assumptions, statistical methods and models can be used to estimate the amount of information already extracted relative to the total amount of information in the complete data. One can then make an informed decision as to whether to continue processing existing samples or collect new ones.
The central difficulty in these types of problems, Meng said, is in finding a procedure sensitive to specifics in particular problems yet general enough to be suitable for software used in a variety of situations.
Monte Carlo simulations
Another area that Meng has worked in extensively is computer simulation.
He is currently developing a method called Monte Carlo integration, which is a way to approximate mathematical quantities – some summing an infinite number of terms – that cannot be calculated exactly no matter how smart one is and how powerful a computer is being used.
These complex problems appear in almost any scientific study where mathematical modeling is important, including those involving protein folding or in modeling atomic bomb blasts.
The method has two steps. The first step – known as a design problem – is to design a method that can generate data that can be used to estimate the desired answer. The second step – known as an inference problem – applies a statistical method to the computer-generated data to approximate the answer, along with its associated statistical errors.
Meng, who has made important contributions to both areas, for years was troubled by the relative unsophistication of the techniques used in the inference area compared with the maturity of techniques and thinking used on real-world problems. With a bit of tweaking, he thought, the Monte Carlo approximations could be made more accurate without increasing the cost or size of the simulations.
After several years of research with several of his colleagues at the University of Chicago, Meng thinks he’s hit on the reason why the analyses of Monte Carlo-generated data pales compared with real-world analyses: missing information.
Or rather, no missing information.
In any real-world inference problem, certain things are unknown and statistical methods have been developed to figure out those unknowns from the collected data. The problem with the computer simulations is that nothing is unknown because the model that generates the simulated data is completely known to the investigator.
Therefore, to apply statistical methods developed for the real world to the Monte Carlo’s simulated data, one has to ignore part of the knowledge of the simulation model, Meng said.
“With real data, we always have to make inferences from what’s known to what’s unknown,” Meng said. “In Monte Carlo integration, treating everything as known is an illusion because we cannot use all of our knowledge in coming up with an answer. The message we’re trying to get out is we have done a fantastic job creating these powerful Monte Carlo algorithms but we’re doing a poor job analyzing them once we have the data.”
Meng said they have developed a general method to decide which knowledge should be ignored, something called “baseline measure.” Meng said once they hit on this idea he instantly realized that this is the “missing link” because the baseline measure’s infinitely many unknowns reflect the fact that Monte Carlo integration’s errors are because no computer can enumerate infinitely many possibilities.
Meng said he’s excited about this finding, particularly because the theory and methods have worked out so beautifully so far, revealing wonderful new insights.
Studying randomness
Meng, a native of Shanghai, received a bachelor of science degree in 1982, then went on to receive a diploma in graduate study of mathematical statistics from Fudan University in 1986. After that, he applied for graduate school at Harvard and said he was surprised when he was accepted, especially with a full scholarship.
“At the time of applying, a lot of people, myself included, thought I was joking, since Harvard was and perhaps still is simply unreachable and unaffordable in many Chinese students’ minds,” Meng said. “I am certainly very grateful to Harvard for its full fellowship, which made it possible for me to pursue my fascination in this most stimulating university.”
He received a master of arts in statistics from Harvard in 1987 and a doctorate in statistics in 1990. After graduating from Harvard, Meng went on to teach at the University of Chicago, becoming an assistant professor in 1991, an associate professor in 1997 and professor in 2000.
Rubin, who served as Meng’s adviser when Meng was a graduate student here, said Meng was very strong technically when he arrived at Harvard and proceeded to develop an intuitive sense of statistics that many never do.
“He’s so successful, it’s hard to say I anticipated that when he first came,” Rubin said. “Some people get that intuition and some people don’t, and he did so spectacularly.”